Today, communication has evolved far beyond traditional methods, paving the way for innovative technologies like Conversational AI. From Siri scheduling your meetings to chatbots guiding you through customer service inquiries, it is transforming the way we interact. But what exactly is Conversational AI, and how does it seamlessly simulate human-like conversations?
In this blog, we will delve into the intricacies of Conversational AI, exploring its underlying technologies, practical applications, and its ability to understand and respond to human language. To gain a better understanding of the differences between Chatbots and Conversational AI, and how they contribute to transforming customer interactions, check out the full article.
What is Conversational AI?
Conversational AI refers to a subset of artificial intelligence designed to facilitate human-like interactions between users and technology. It powers systems such as chatbots, virtual assistants, and voice-activated devices to simulate real-time conversations through natural language processing (NLP) and machine learning (ML) capabilities.
Conversational AI combines multiple technologies, including automated speech recognition (ASR), natural language understanding (NLU), and text-to-speech (TTS) processing, to interpret, respond, and adapt to user inputs effectively. Unlike traditional chatbots, which rely on scripted responses, Conversational AI for SaaS Products systems are dynamic, capable of learning and improving over time, offering more personalized and context-aware responses.
Why is Conversational AI Important?
The importance of conversational AI lies in its transformative impact on customer interactions, operational efficiency, and user experience. Here’s why it is critical across various domains:
1. Enhanced Customer Experience
Conversational AI enables businesses to deliver seamless, 24/7 customer support. By understanding user intent and providing contextually relevant responses, it reduces wait times and enhances satisfaction, ensuring consistent engagement across channels.
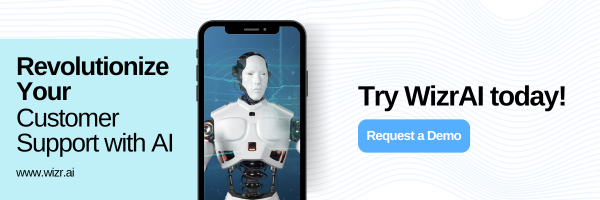
2. Increased Operational Efficiency
By automating repetitive tasks, such as answering FAQs or managing routine customer queries, conversational AI reduces the workload on human agents. This allows teams to focus on more complex and value-driven tasks, increasing overall efficiency.
3. Scalability
Traditional customer support systems face scalability challenges as businesses grow. Conversational AI scales effortlessly, handling a high volume of queries simultaneously without compromising performance or accuracy, making it ideal for growing enterprises.
4. Cost-Effectiveness
Adopting conversational AI can significantly lower operational costs by minimizing the need for extensive human resources. Over time, the system’s ability to learn and adapt improves its efficiency, offering long-term cost benefits.
5. Multilingual and Omnichannel Support
Conversational AI solutions can interact with users in multiple languages and integrate across various platforms, such as websites, mobile apps, and messaging platforms. This capability makes them indispensable for global businesses aiming to provide a seamless omnichannel customer support experience and engage diverse audiences effectively.
6. Personalization
Through data analysis and machine learning, conversational AI tailors interactions to individual users. It remembers past interactions, learns preferences, and anticipates needs, creating a personalized and meaningful user experience.
What Are the Key Components of Conversational AI Platforms?
Conversational AI is a sophisticated blend of various technologies working in harmony to enable machines to understand, process, and respond to human language. Here are the key components that make up the foundation of Conversational AI:
1. Natural Language Processing (NLP)
Natural Language Processing (NLP) is the cornerstone of Conversational AI, enabling machines to interpret and generate human language. It involves several sub-components:
- Tokenization: Breaking down text into individual words or phrases. It helps in understanding the structure and meaning of the text, making it easier to analyze and process.
- Part-of-Speech Tagging: Identifying the grammatical components of each word, such as nouns, verbs, adjectives, and adverbs. This helps the AI understand the roles and relationships of words in a sentence.
- Named Entity Recognition (NER): Detecting specific entities like names, dates, and locations within text. NER is crucial for extracting relevant information and providing accurate responses.
- Sentiment Analysis: Determining the emotional tone behind words. Sentiment analysis allows the AI to gauge the user’s mood and respond appropriately, enhancing user experience and engagement.
2. Machine Learning (ML)
Machine learning algorithms are crucial for training Conversational AI models. By learning from vast datasets, these models improve their ability to understand and generate human-like responses over time. ML techniques used in Conversational AI include:
- Supervised Learning: Training models on labeled data to predict outputs. Supervised learning helps the AI learn from examples and make accurate predictions based on new data.
- Unsupervised Learning: Identifying patterns and structures in unlabeled data. Unsupervised learning is useful for discovering hidden patterns and insights within the data without predefined labels.
- Reinforcement Learning: Improving models through trial and error, based on feedback. Reinforcement learning enables the AI to learn optimal actions through rewards and penalties, improving its decision-making capabilities.
3. Speech Recognition
This component converts spoken language into text. It’s essential for voice-activated AI systems like virtual assistants. Speech recognition involves voice call sentiment analysis, which plays a crucial role in understanding customer emotions and feedback during interactions.
- Acoustic Modeling: Mapping audio signals to linguistic units. Acoustic modeling helps the AI recognize different sounds and convert them into corresponding words.
- Language Modeling: Predicting word sequences to improve accuracy. Language modeling helps the AI understand the context and predict the most likely next words in a sequence, enhancing recognition accuracy.
4. Dialogue Management
Dialogue management orchestrates the flow of conversation. It decides how the system should respond based on the user’s input and the context of the conversation. Key functions include:
- State Tracking: Keeping track of the conversation’s context and user intent. State tracking ensures that the AI maintains context throughout the interaction, providing relevant and coherent responses.
- Policy Learning: Determining the best response strategy based on the current state and historical interactions. Policy learning helps the AI adapt its responses to achieve specific goals, such as resolving user queries efficiently.
5. Natural Language Generation (NLG)
NLG is the process of producing coherent and contextually appropriate text or speech responses. It involves:
- Content Determination: Deciding what information to convey. Content determination ensures that the AI provides relevant and useful information in its responses.
- Sentence Planning: Structuring sentences for clarity and coherence. Sentence planning helps the AI construct grammatically correct and logically ordered sentences.
- Surface Realization: Converting structured sentences into grammatically correct text. Surface realization ensures that the AI’s responses are natural and easy to understand.
6. Context Management
Understanding context is vital for maintaining coherent conversations. Context management systems track past interactions and user preferences to provide relevant and personalized responses. This includes:
- Contextual Awareness: Recognizing and remembering information from previous interactions to maintain continuity.
- User Profiling: Storing and utilizing user-specific information, such as preferences and history, to tailor responses.
7. Integration with External Systems
Conversational AI often needs to interact with other systems to fetch information or perform tasks. Integration with databases, APIs, and other enterprise systems ensures that the AI can provide accurate and up-to-date responses. This integration allows:
- Real-Time Data Access: Fetching current information to answer user queries accurately.
- Task Automation: Performing actions such as booking appointments, processing orders, or retrieving account details seamlessly.
8. User Interface (UI)
The user interface is how users interact with the AI, whether through text, voice, or visual means. A well-designed UI ensures a seamless and intuitive user experience. Key aspects include:
- Usability: Ensuring the interface is easy to navigate and use.
- Accessibility: Making the AI accessible to users with different needs and preferences, including those with disabilities.
- Visual Design: Creating an appealing and engaging interface that enhances the overall user experience.
By combining these components, Conversational AI systems can deliver highly interactive and human-like conversational experiences, revolutionizing the way we interact with technology.
How Do Conversational AI Platforms Work?
Conversational AI is a marvel of modern technology, integrating several advanced processes to create seamless and human-like interactions. Here’s a step-by-step breakdown of how it works:
1. User Input
The interaction begins when the user inputs a query or command. This can be through text, such as typing a question into a chatbot, or through speech, such as speaking to a virtual assistant like Siri or Alexa.
2. Speech Recognition
If the input is in spoken form, the system uses Automatic Speech Recognition (ASR) to convert the audio into text. ASR systems utilize acoustic models to understand spoken words and language models to predict word sequences, ensuring accurate transcription, which is crucial for effective voice call sentiment analysis in contact centers.
3. Natural Language Processing (NLP)
Once the input is in text form, NLP comes into play. NLP is divided into several stages:
- Tokenization: Breaking down the text into individual words or tokens.
- Part-of-Speech Tagging: Identifying the grammatical roles of each word (nouns, verbs, adjectives, etc.).
- Named Entity Recognition (NER): Extracting specific entities such as names, dates, and locations.
- Dependency Parsing: Analyzing grammatical structure to understand relationships between words.
- Sentiment Analysis: Determining the emotional tone of the message to provide contextually appropriate responses.
4. Intent Recognition and Context Understanding
The system uses intent recognition to determine what the user wants to achieve with their input. This involves analyzing the text to identify the user’s intent, such as booking a flight, checking the weather, or troubleshooting an issue. Context understanding helps maintain the flow of conversation by remembering previous interactions and user-specific information.
5. Dialogue Management
Dialogue management is responsible for orchestrating the conversation. It keeps track of the context and state of the conversation, ensuring that responses are coherent and relevant. This involves:
- State Tracking: Monitoring the conversation’s progress and the user’s intent.
- Policy Learning: Deciding the best course of action based on current context and historical interactions.
6. Backend Integration
To provide accurate and useful responses, Conversational AI systems often need to access external data sources or perform specific actions. This involves:
- Database Queries: Fetching relevant information from databases, such as retrieving user account details or product information.
- API Calls: Interacting with external services to perform tasks, such as booking appointments, placing orders, or fetching live data like weather updates or stock prices.
7. Natural Language Generation (NLG)
Once the necessary information is gathered, the system generates a response using NLG. This involves:
- Content Determination: Deciding what information to include in the response.
- Sentence Planning: Structuring the information into coherent sentences.
- Surface Realization: Ensuring the response is grammatically correct and natural-sounding.
8. Output Delivery
The generated response is then delivered to the user. If the interaction is through a text-based interface, the response is displayed on the screen. If it’s a voice interaction, the text response is converted back into speech using Text-to-Speech (TTS) technology, which synthesizes a human-like voice to relay the response.
9. Learning and Improvement
Conversational AI systems continuously learn and improve from user interactions. Machine learning algorithms analyze past conversations to enhance accuracy and effectiveness. Feedback loops and reinforcement learning help the system adapt to user preferences and refine its responses over time.
Real-World Applications of Conversational AI Across Industries
Conversational AI has transcended the realm of futuristic technology and become an integral part of our daily lives. Its versatility and efficiency are being harnessed across various industries to enhance user experiences, streamline operations, and provide innovative solutions. For SaaS companies, understanding the difference between Conversational AI vs. Generative AI is crucial to determine which technology best suits their needs and use cases. Here’s a look at some of the most impactful real-world applications of Conversational AI:
1. Customer Service
Conversational AI is revolutionizing customer service by providing instant, 24/7 support. Here’s how:
- Chatbots: Many companies use AI-powered chatbots on their websites to handle common queries, guide users through processes, and resolve issues without human intervention. This reduces wait times and improves customer satisfaction.
- Virtual Agents: More advanced systems, like virtual agents, can understand complex queries and provide personalized responses. They can handle a variety of tasks, from troubleshooting technical issues to processing returns and refunds.
2. Virtual Assistants
Virtual assistants like Amazon’s Alexa, Apple’s Siri, Google Assistant, and Microsoft’s Cortana are some of the most well-known Conversational AI. They help users by:
- Managing Daily Tasks: Setting reminders, alarms, and calendar events.
- Providing Information: Answering questions, checking the weather, and offering news updates.
- Controlling Smart Devices: Managing smart home devices like lights, thermostats, and security systems.
3. E-commerce and Retail
In the retail sector, Conversational AI enhances the shopping experience and boosts sales by:
- Personalized Shopping Assistance: AI chatbots can recommend products based on user preferences, previous purchases, and browsing history.
- Order Tracking: Customers can easily check the status of their orders and receive updates via chatbots.
- Customer Engagement: Interactive chatbots can engage users with promotions, product launches, and exclusive deals, enhancing customer loyalty.
4. Healthcare
Conversational AI is making significant strides in healthcare by improving access to information and services:
- Symptom Checking: AI chatbots can assess symptoms and suggest possible conditions or actions, helping users decide whether to seek medical attention.
- Appointment Scheduling: Patients can book, reschedule, or cancel appointments through conversational interfaces, reducing administrative workload.
- Mental Health Support: AI-driven platforms provide immediate mental health support, offering coping strategies and connecting users to professional help if needed.
5. Banking and Finance
In the banking sector, Conversational AI streamlines operations and improves customer experiences by:
- Account Management: Chatbots help users check account balances, view transaction histories, and transfer funds.
- Fraud Detection: AI systems can monitor transactions for unusual activity and alert users to potential fraud.
- Financial Advice: Virtual financial advisors provide investment tips, savings strategies, and personalized financial planning.
6. Education
Educational institutions and platforms are leveraging Conversational AI to enhance learning experiences:
- Tutoring and Assistance: AI tutors provide personalized learning assistance, helping students with homework and understanding complex subjects.
- Administrative Support: Chatbots help students with registration, course selection, and accessing academic resources.
- Language Learning: AI-driven language learning apps use conversational interfaces to practice speaking and understanding new languages.
7. Human Resources
In HR, Conversational AI simplifies and automates many processes:
- Recruitment: AI chatbots can pre-screen candidates, schedule interviews, and answer questions about job openings.
- Employee Onboarding: New employees can use chatbots to get information about company policies, benefits, and training programs.
- Employee Support: AI assistants provide quick answers to HR-related queries, such as leave balances and payroll information.
8. Travel and Hospitality
The travel industry benefits from Conversational AI by enhancing customer service and personalizing travel experiences:
- Booking and Reservations: Chatbots assist users in finding flights, hotels, and rental cars, making bookings, and managing reservations.
- Travel Assistance: Virtual assistants provide information about destinations, weather, local attractions, and itineraries.
- Customer Support: AI chatbots handle inquiries about travel policies, cancellations, and refunds, ensuring smooth customer experiences.
Conclusion
Conversational AI represents a significant advancement in the way humans interact with technology. By leveraging sophisticated techniques such as natural language processing, machine learning, and deep learning, these systems can understand and respond to human language with remarkable accuracy. From virtual assistants to customer service bots, Conversational AI for SaaS Products is transforming industries by enhancing user experiences and streamlining operations. As technology continues to evolve, the potential applications of Conversational AI will only expand, making it an integral part of our digital landscape.
About Wizr AI
Wizr AI is an Advanced Enterprise AI Platform that empowers businesses to build Autonomous AI Agents, AI Assistants, and AI Workflows, enhancing enterprise productivity and customer experiences. Our CX Control Room leverages Generative AI to analyze insights, predict escalations, and optimize workflows. CX Agent Assist AI delivers Real-Time Agent Assist, boosting efficiency and resolution speed, while CX AutoSolve AI automates issue resolution with AI-Driven Customer Service Automation. Wizr Enterprise AI Platform enables seamless Enterprise AI Workflow Automation, integrating with data to build, train, and deploy AI agents, assistants, and applications securely and efficiently. It offers pre-built AI Agents for Enterprise across Sales & Marketing, Customer Support, HR, ITSM, domain-specific operations, Document Processing, and Finance.
Experience the future of enterprise productivity—request a demo of Wizr AI today.
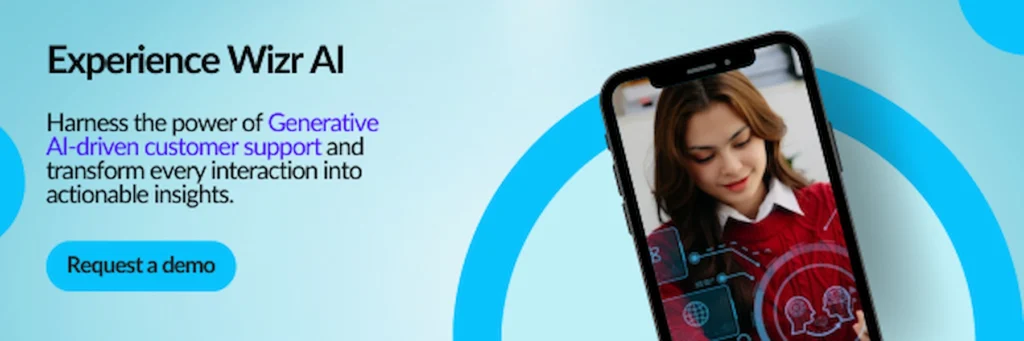