There has been significant progress in artificial intelligence over the past decade. Two important technologies that have emerged during this period are Generative AI and Natural Language Processing (NLP). Natural Language Processing (NLP) in Generative AI relies on NLP to create different types of content, such as text and images. NLP in Generative AI acts as a mediator between computers and human language, giving them the ability to tackle complex linguistic frameworks. In this blog, we will examine the role of NLP in Generative AI and the interaction between generative models and Natural Language Processing (NLP) in Generative AI, allowing them to transform language-based applications and enhance AI’s human-like speech comprehension and production capabilities.
What is Natural Language Processing (NLP)?
Natural Language Processing (NLP) is a branch of artificial intelligence (AI) that looks into the interaction between computers and humans to enable machines to comprehend what we say or write and help them generate grammatically correct information with meaning. Essentially, Natural Language Processing (NLP) in Generative AI uses computational linguistic techniques integrated with machine learning methods to process data found in texts, allowing AI to, for example, translate one language into another or express sentiments about written content, such as emails, using computer-generated voices. This capability helps identify genuine meanings rather than merely surface signals from people speaking over the telephone, even if they do not sound like native speakers, for instance.
The Role of Natural Language Processing (NLP) in Generative AI
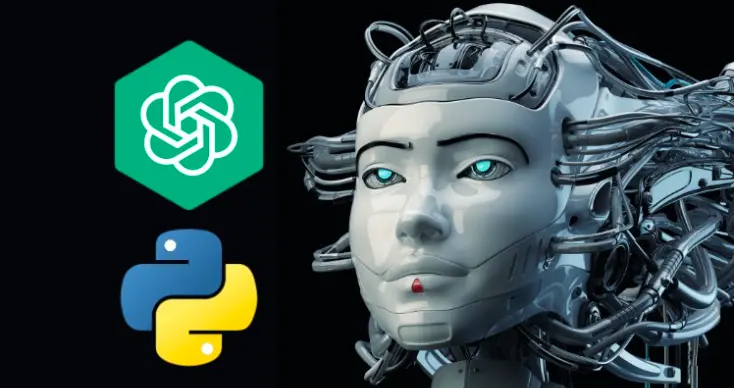
Generative AI models, such as GPT, ensure significant levels of coherence in written and contextually related information. However, Natural Language Processing (NLP) in Generative AI plays a crucial role in understanding the subtleties of spoken or written language within these models. NLP Applications in Generative AI enhance generative AI capabilities by providing the necessary tools for understanding user inputs and processing natural human language to generate relevant responses or produce content that reflects that input.
NLP vs. Generative AI: Key Differences show how Natural Language Processing (NLP) in Generative AI enables the system to recognize users’ intentions, retain context, and provide accurate responses when interacting with a generative AI chatbot. Additionally, NLP in Generative AI allows these models to go beyond basic text generation tasks, such as summarizing lengthy essays, categorizing articles based on their content, or determining sentiment. This advanced NLP, combined with generative features, helps AI prevent the creation of irrelevant sentences that may seem out of place, thereby delivering responses that sound more like those of real humans.
Natural Language Processing (NLP) vs Generative AI : Key Differences
Generative AI and Natural Language Processing (NLP) are both crucial for modern AI applications. However, they play different roles in the AI ecosystem. Generative AI aims to generate new data, like text, images, or sound, from input currently available or learned patterns. It can also construct answers that resemble those made by people or even create artwork that never existed before. Models like GPT (Generative Pre-trained Transformers) exemplify Generative AI and Natural Language Processing (NLP), showcasing the ability to write meaningful, relevant text based on its data.
Unlike Generative AI, whose main objective is the generation of information, NLP (Natural Language Processing) handles the understanding and processing of human language. This means that rather than producing something entirely new from scratch, Natural Language Processing (NLP) in Generative AI is more focused on interpreting, analyzing, and responding to human-like sentences. Text classification, sentiment analysis, and language translation are some of the tasks encompassed by NLP Applications in Generative AI.
The primary difference between these two technologies lies in their fundamental goals: while Generative AI creates content, NLP makes that content understandable or interpretable by humans. Together, these two work seamlessly, with NLP enhancing Generative AI by assisting in interpreting language inputs, allowing Generative AI to leverage this understanding to generate meaningful outputs.
How NLP Enhances Generative AI Capabilities
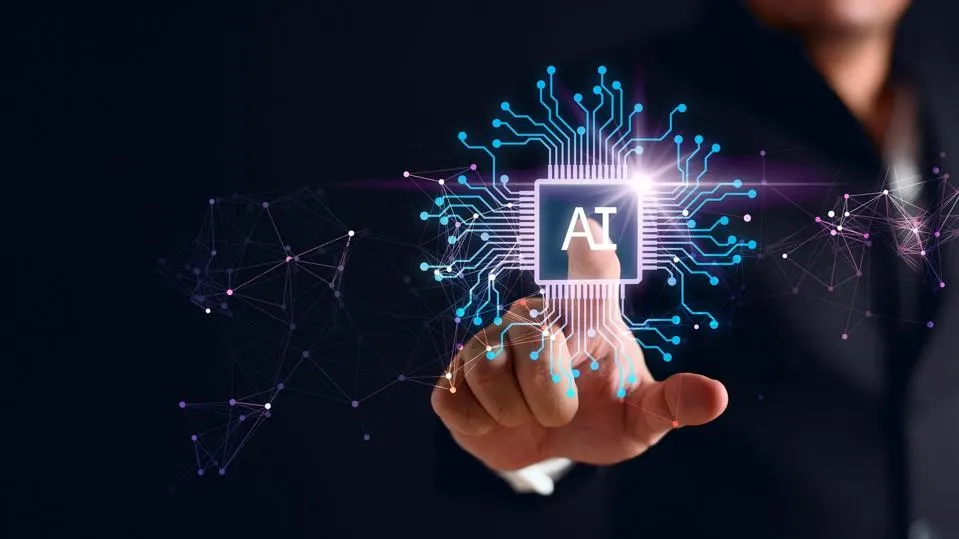
Natural Language Processing (NLP) in Generative AI plays a crucial role in enhancing Generative AI’s ability to produce coherent, contextually aware, and meaningful language-based content. This capability cannot be realized without NLP, as it aids in translating human language and generating responses that align with user expectations.
NLP enhances Generative AI in several critical ways:
- Contextual Understanding: NLP in Generative AI enables the system to examine and comprehend the context of any input it receives. As a result, Generative AI and Natural Language Processing (NLP) work together to provide responses that are consistent with the context and meaning of the words used, whether it involves identifying terms in an article or interpreting sentiments expressed during a discussion.
- Grammatical Accuracy: Natural Language Processing (NLP) enhances Generative AI’s ability to produce grammatically correct content. Additionally, it helps Generative AI create articles that are syntactically sound. NLP Techniques in Generative AI assist in generating content that is fluent and readable in a human-like manner.
- Sentiment and Intent Recognition: One way NLP enhances Generative AI is by detecting users’ moods and intentions, allowing it to adjust its responses accordingly. Natural Language Processing for Customer Sentiment Analysis in AI enables Generative AI to recognize sentiments like anger, allowing the system to respond with empathy or a solution-oriented approach.
- Complex Task Support: NLP Applications in Generative AI also enable Generative AI to perform more complex operations, such as text summarization with NLP in Generative AI, answering technical questions, or translating documents. With its profound understanding of language structure, Generative AI can engage in high-value tasks that go beyond simple text production.
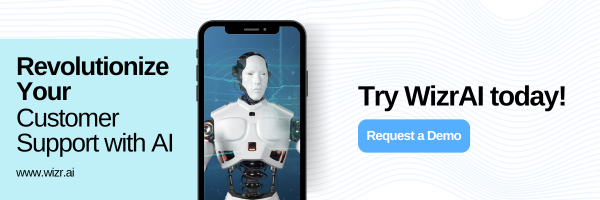
Natural Language Processing (NLP) Techniques Powered by Generative AI
Natural Language Processing (NLP) in Generative AI plays a significant role in enhancing the way Generative AI functions. By combining NLP techniques in Generative AI, it not only produces human-like content but also understands and processes how language works in detail. Below are some important Natural Language Processing (NLP) examples in Generative AI:
Tokenization:
Tokenization breaks down large pieces of text into smaller units, such as words or subwords, which makes it easier for the AI to understand language. This is essential for the role of NLP in Generative AI since Generative AI can take in inputs more effectively and create text that flows logically.
Named Entity Recognition (NER):
NER supports AI systems in recognizing and classifying important information, such as names of persons, places, organizations, or time in a text. By employing Natural Language Processing (NLP) techniques in Generative AI, the system can make more accurate responses based on these recognized entities.
Part-of-Speech (POS) Tagging:
POS tagging helps in identifying the grammatical roles words play in sentences, such as nouns, verbs, and adjectives. This technique is useful for Generative AI and NLP techniques to ensure that generated texts remain grammatical throughout, resulting in more natural and readable outputs.
Dependency Parsing:
Dependency parsing enables the system to analyze the grammatical structure of sentences, revealing the relationships between words. This technique contributes to the role of Generative AI in Natural Language Processing by allowing the AI to understand complex sentence structures and produce coherent content.
Text Summarization:
Through extractive or abstractive summarization methods, NLP applications in Generative AI help reduce long essays into shorter forms while preserving their main points. Text Summarization with NLP in Generative AI is especially useful for applications like news summarization and content distillation.
Text Generation Models:
Generative AI and Natural Language Processing (NLP) rely on models like GPT (Generative Pre-trained Transformers), which are trained on large amounts of data to produce high-quality text. These models can predict the next word or phrase in a sentence by employing Natural Language Generation with AI and complex NLP technologies, such as language modeling, ensuring coherence in the generated text.
NLP techniques in Generative AI enhance the system’s ability to process language inputs effectively, leading to outputs of higher complexity and sensitivity to related ideas. These techniques demonstrate the role of NLP in Generative AI and the practical applications of NLP in Artificial Intelligence, enabling more advanced and accurate content generation.
Sentiment Analysis in Artificial Intelligence (AI) Using Natural Language Processing (NLP)
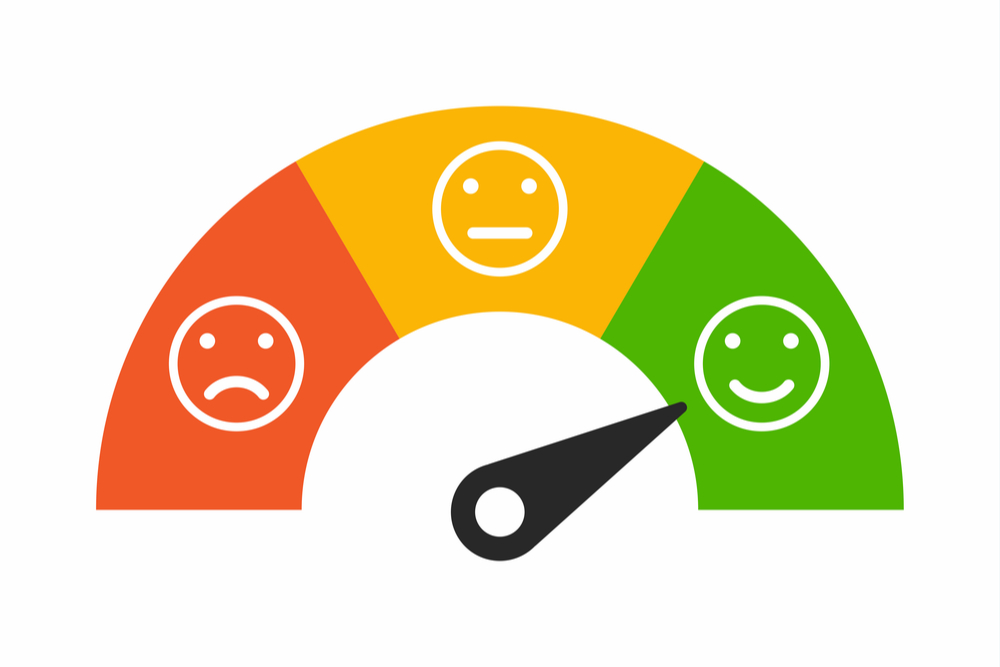
Natural Language Processing (NLP) in Generative AI plays a critical role in customer sentiment analysis within Artificial Intelligence (AI), enabling machines to understand and interpret the emotional tone behind text. By incorporating NLP techniques in Generative AI, machines can conduct opinion mining, determining whether a text is positive, negative, or neutral, especially in contexts like customer reviews, social media posts, or user interactions with AI agents.
Here’s how NLP enhances the sentiment analysis process within AI:
- Emotion Detection: Natural Language Processing (NLP) in Generative AI techniques allow AI systems to go beyond mere problem identification by detecting underlying emotions. This capability highlights the role of NLP in Generative AI and how it aids in understanding human sentiment.
- Text Classification with NLP: Through Natural Language Processing text classification in AI, machines categorize sentence meanings based on sentiment. This process is crucial for applications like customer feedback analysis and product review systems, where NLP applications in Generative AI help train models to identify sentiments as negative, positive, or neutral.
- Contextual Sentiment Analysis: Generative AI and Natural Language Processing (NLP) together enable AI to interpret complex speech patterns, such as sarcasm or irony. For instance, in the sentence, “This product is surprisingly good,” NLP techniques in Generative AI help identify the positive sentiment by recognizing the word “surprisingly” as a shift in meaning. This showcases the applications of NLP in artificial intelligence for deeper contextual understanding.
- Aspect-Based Sentiment Analysis: NLP applications in Generative AI allow for the breakdown of text into various components, enabling AI to evaluate different sentiments expressed within a single piece of writing. For example, a review might praise customer service while criticizing product quality. With Natural Language Processing (NLP) in Generative AI, AI can assess each sentiment separately, showcasing the role of Generative AI in natural language processing.
- Real-Time Feedback with NLP: Natural Language Processing for sentiment analysis in AI is essential for real-time customer service applications. By using Generative NLP techniques and applications, AI can gauge customer emotions during interactions and adjust responses accordingly, improving customer satisfaction and preventing frustration.
Practical Applications of Natural Language Processing (NLP) in Generative AI
The practical applications of Natural Language Processing (NLP) in Generative AI are expanded by NLP, in which AI can mimic the language used by humans very accurately. Taking advantage of NLP, there is a fusion between this field and Generative AI, leading to the generation of several types of applications that are present in real life across various sectors, thus changing how people relate to systems driven by artificial intelligence. Here are some important practical uses of NLP Applications in Generative AI within interactive language systems powered by generative AI:
Conversational AI Assisting Tools
These chatbots are based on principles from Natural Language Processing (NLP) in Generative AI, allowing them to converse like us—examples include Siri, Alexa, and various customer assistants. These chatbots leverage NLP by processing language input from users, ultimately allowing them to provide instant feedback. By using pre-built models from the machine learning toolbox, a chatbot trained with Generative AI and NLP Techniques could perform sentence completions via composition to avoid monotony.
Content Creation & Copywriting
Content creation has been revolutionized through the combined use of Generative AI technology and NLP in generating articles, reports, and even marketing copy. Journalism is one profession that widely benefits from this technology due to the ability to produce written materials quickly and efficiently through auto-generated article drafts. This highlights the role of generative AI in Natural Language Processing.
Automatic Summarization
This involves using NLP Techniques in Generative AI such as extractive and abstractive summarizations, allowing generative AIs to condense large data loads into brief summaries. Examples include legal work, where lawyers may need summaries of lengthy court cases, and the medical field, where scientific research papers can have thousands of words each. If you don’t want your readers to spend hours sifting through documents for the information they require on just one page, ensure that your content is easily digestible. This process can be categorized under Text Summarization with NLP in Generative AI.
Machine Translation
Through the integration of Natural Language Processing (NLP) in Generative AI, significant strides have been made in improving machine translations, ensuring that they are more accurate and natural across various target languages. One such example is Google Translate, which employs NLP Techniques in Generative AI to break down complex language structures for translations that are as close as possible in meaning and tone to the original. By grasping finer points of grammar, syntax, and cultural background, it becomes evident that these computer programs are improving daily at providing real-time, top-quality translations.
Sentiment Analysis & Content Moderation
Natural Language Processing for Customer Sentiment Analysis in AI helps NLP-based generative AIs understand the feelings behind user-generated content in applications like social media monitoring and customer feedback analysis. AI models have the capability to determine whether an article or comment is written from a positive or negative point of view, thereby enabling companies to assess customer sentiment or moderate any inappropriate content. NLP-backed generative AIs can also interact with users by responding to their comments, thereby addressing complaints or supporting and encouraging feedback.
Text-to-Speech (TTS) & Speech-to-Text (STT)
Generative AI platforms are powered by NLP for TTS and STT conversion, making it easier to communicate through natural language processing. Text-to-speech systems, commonly used in accessibility devices, allow for the conversion of written information into spoken language, while speech recognition tools accurately convert voice messages, such as transcribing software applications and voice-controlled assistants that rush to capture each spoken word. Thus, facilitating dialogue between those who need help and others who may not yet know how to write. An example of this could be when someone tweets for help on Twitter but cannot type because they are injured or unable to use a keyboard. Voice call sentiment analysis also plays a crucial role in ensuring a seamless customer service experience through real-time voice recognition and emotional understanding.
Recommendation Engines
NLP Applications in Generative AI analyze user behavior and preferences and are found in e-commerce recommendation engines, entertainment platforms, and digital content. By processing natural language inputs, such as reviews, search queries, or social media interactions, AI can generate personalized recommendations for products, services, or content that users would enjoy the most. Netflix and Spotify use this technology to suggest films or songs based on past behavior and tastes of their customers.
Customer Service Automation
Natural Language Processing (NLP) in Generative AI enables generative AI in customer support automation to receive and answer customer inquiries accurately without the need for human intervention. Ticket routing within AI, automatic troubleshooting, and individualized support all require the interpretation of complex phrases enabled by NLP, leading to quicker problem resolution times while also improving clients’ experiences. This approach also reduces employee workloads at contact centers.
Conclusion
The fusion of NLP and Generative AI is fundamentally transforming how humans interact with computers. NLP serves as a foundation for machines to understand, analyze, or generate spoken or written language, while Generative AI takes this a step further by creating novel, well-structured text that resembles human output. These changes are evident across various sectors, from business-customer relations to content generation, ensuring tailored communication. As both technologies continue to evolve, their integration will pave the way for even more innovative applications, leading artificial intelligence into new horizons. This emphasizes the NLP vs. Generative AI: Key Differences and the importance of understanding their synergy.
About Wizr AI
Wizr enhances customer support seamlessly with AI-powered customer service tools. Cx Hub predicts and prevents escalations, while Agent Assist boosts productivity with automated customer service software. Auto Solve handles up to 45% of tickets, freeing agents for complex issues. Cx Control Room analyzes sentiment to guide proactive solutions, maximizing satisfaction and retention. Guided by generative AI for customer support, Wizr prioritizes exceptional customer experiences. To explore how these tools can benefit your business, request a demo of Wizr AI today.
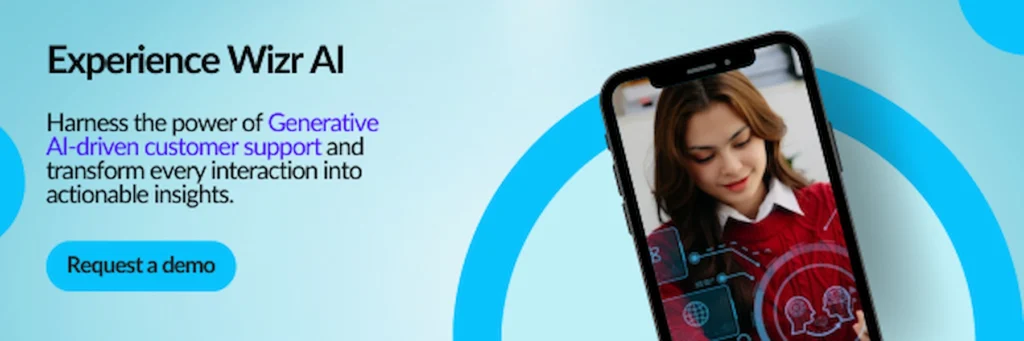
AI Workflow Builder: Transforming Business Automation in 2025
In 2025, businesses are in the process of changing their operational methods by leveraging the potential that AI Workflow Builders…
Voicebot vs Chatbot: Which AI is Best for Your Customer
Introduction Customer support is undergoing a revolution with the rise of artificial intelligence (AI). A recent study revealed that 67% of…
How to Build an FAQ Chatbot: Examples, Types & Benefits
Introduction The first recorded prototype of today’s chatbot is ‘Eliza,’ created in the late 1900s. Moving forward to Siri and Alexa,…
AI Text Classification: What It Is & How It Boosts
One game-changing technology currently transforming how industries manage communication with customers, including after-sales services, is AI Text Classification. Reporting helps…
Generative AI in B2B SaaS: Unlocking the Future of Smarter
Introduction Imagine a world where every customer query is resolved instantly, every support interaction feels personal, and operational costs plummet without…
AI Voice Chatbots: How They Work and Why They Matter
Introduction AI Voice Chatbots are transforming the way businesses interact with customers. Combining advanced AI voice recognition chatbots technologies with artificial…