Businesses seeking to improve their products and services need to analyze customer feedback, but traditional methods often fail to capture what consumers truly think. NLP Sentiment Analysis for Customer Feedback is a modern tool that helps companies better understand customer feedback. Specifically, Natural Language Processing Sentiment Analysis enables businesses to interpret client feedback using NLP-based tools without the need for biased responses or face-to-face interviews.
This technology relies on sophisticated NLP Algorithms for Sentiment Analysis and models to go beyond surface-level text and identify emotions, opinions, and hidden patterns in data. By detecting satisfaction levels in product reviews or identifying anger in support tickets, Real-Time Sentiment Analysis with NLP for Customer Feedback provides actionable insights into customer sentiment, enabling organizations to make customer-focused decisions based on up-to-date information. This blog post will explore the challenges of Sentiment Analysis in NLP and why it is essential for businesses to understand customer feedback today.
Why is Natural Language Processing (NLP) Essential for Feedback Analysis?
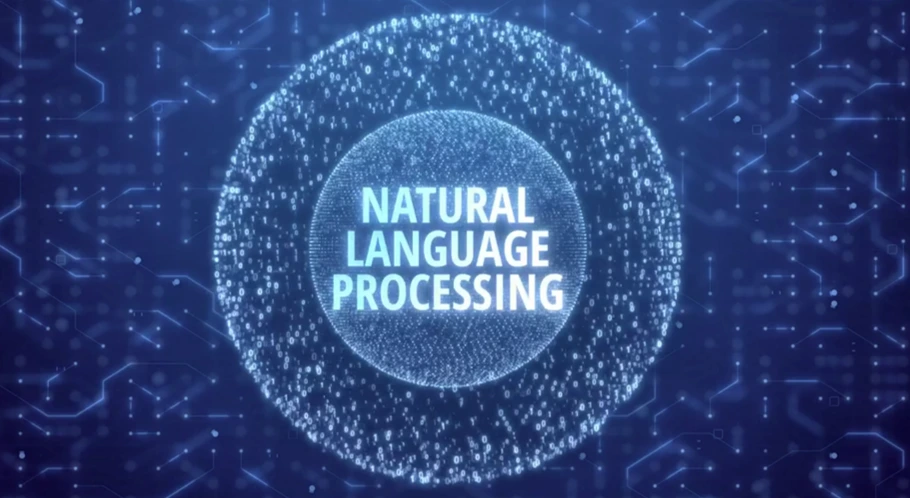
Customer feedback is present across various channels, including emails, social media, reviews, and surveys, making its way into today’s digital landscape. Unfortunately, manually analyzing this data is impractical and error-prone due to its unstructured nature and sheer volume. This is where NLP for Customer Feedback Analysis comes into play. NLP Text Analytics enables machines to comprehend, interpret, and analyze human language, simplifying the process of extracting valuable insights from customers’ opinions.
With Text Mining for Customer Feedback Sentiment, businesses can identify patterns, spot recurring issues, and understand the true sentiments of their customers. This helps pinpoint key areas needing attention by analyzing thousands of comments posted on websites every day. Advanced Sentiment Analysis for Customer Feedback proves invaluable in reducing the time required for this task. It ensures that companies can respond promptly and effectively, whether addressing issues that are usually hidden or identifying new opportunities from customer feedback. Ultimately, regardless of how complex the language may be or where it originates, the goal is always to enhance the customer experience.
What is Sentiment Analysis and How Does NLP Enhance It?
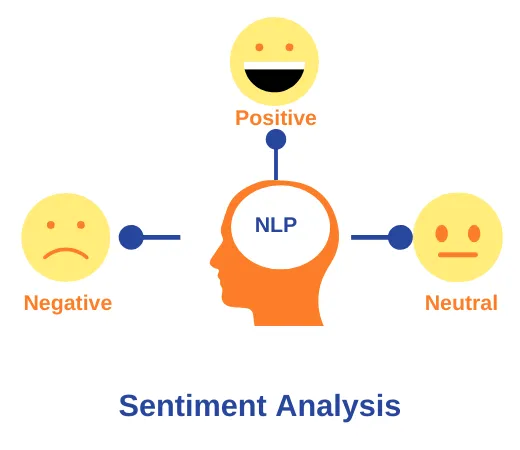
Sentiment Analysis in NLP involves determining the emotions expressed in text, such as positivity, negativity, or neutrality. This helps companies understand what customers think about their products, services, or brand in general. However, traditional sentiment analysis techniques, which rely on simple keyword matching, often overlook the subtleties of language that humans use in speech, such as sarcasm, slang, and context.
At this point, Natural Language Processing in Sentiment Analysis is making significant advances. NLP Models for Sentiment Analysis use complex models, including AI Sentiment Analysis Models and machine learning, to understand the context and tone of feedback. For example, even with similar wording, NLP can distinguish between an irritated complaint and constructive criticism.
Additionally, it can detect emotions, providing deeper insights into the intensity and nature of a customer’s feelings. The improvement of Sentiment Analysis Using NLP for Customer Feedback helps businesses gain more accurate information about customers’ perspectives, leading to actionable findings—such as identifying areas for service improvement or using positive feedback to strengthen customer trust. In simpler terms, NLP makes sentiment analysis both faster and more reliable, offering deeper insights.
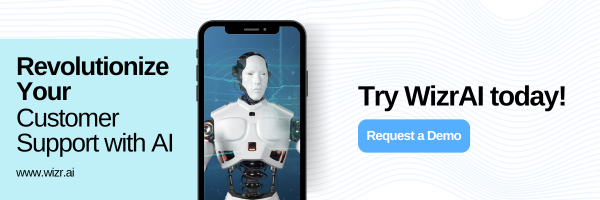
How Does NLP Work in Sentiment Analysis?
NLP Sentiment Analysis for Customer Feedback leverages Natural Language Processing in Sentiment Analysis to transform raw text into structured insights. By applying NLP Models for Sentiment Analysis and advanced NLP Algorithms for Sentiment Analysis, businesses gain a better understanding of customer emotions. Here’s how Sentiment Analysis Using NLP for Customer Feedback works:
Treatment of Text: The first step in Text Mining Using NLP involves cleaning the text by removing irrelevant elements like punctuation marks, special characters, stop words, and extra spaces. This preparation is vital for NLP Text Analytics to deliver accurate results.
Tokenization: After cleaning, texts are divided into smaller components like words or phrases using NLP Models for Text Classification. This process enables efficient analysis of individual units of feedback..
Part-of-Speech (POS) Tagging: This step involves assigning grammatical labels to words, aiding Natural Language Processing Sentiment Analysis in understanding sentence structures. For instance, it helps differentiate “good” as an adjective or a noun in the context of the feedback.
Sentiment Scoring: Using NLP for Sentiment Analysis, models assign sentiment scores to words or phrases. These scores, based on emotional weight, help determine whether feedback is positive, negative, or neutral. Emotion Analysis NLP also identifies complex states such as frustration, happiness, or sadness.
Context Analysis: Advanced NLP Text Mining tools analyze relationships between words to infer subtle meanings. For example, phrases like, “The product is amazing, but the delivery was awful,” are assessed for mixed sentiments through Emotion Detection in NLP.
Aggregation: The final step of Advanced Sentiment Analysis with NLP involves summing up sentiments from individual sentences to determine the overall tone. Whether positive, negative, or neutral, this aggregation is crucial for insights into customer feedback.
Key Benefits of NLP Sentiment Analysis for Customer Feedback
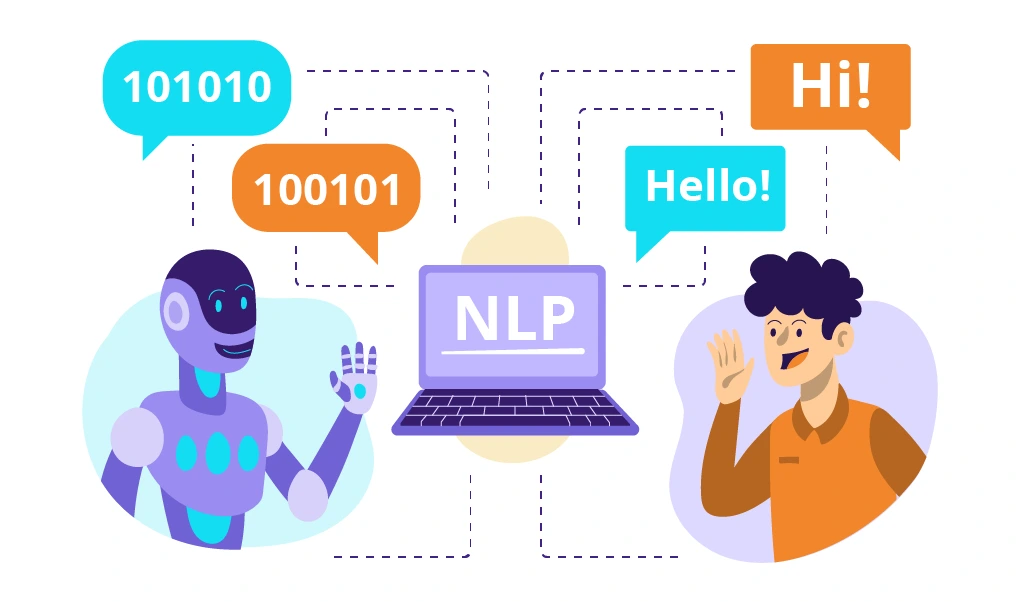
NLP Sentiment Analysis for Customer Feedback provides numerous benefits that significantly change how businesses manage client responses. Below are the main advantages of aligning Natural Language Processing in Sentiment Analysis with customer feedback analysis, from improved accuracy to faster insights:
Scalability
One of the key advantages of NLP Models for Sentiment Analysis is its ability to process large amounts of data at once. This includes processing thousands of social media posts, reviews, or survey responses within seconds, enabling businesses to track sentiment across multiple channels. This ensures that businesses of any size can effectively monitor and respond to customer sentiment in real-time using Text Mining for Customer Feedback Sentiment.
Precision
Traditional approaches to sentiment analysis usually rely on basic keyword detection, which can wrongly interpret the context or tone of communication. In contrast, NLP for Sentiment Analysis digs deeper to understand what lies beneath the words, including contextual understanding, mood awareness, and nuances in customer language. This comprehension facilitates the correct classification of emotions, allowing businesses to make better decisions about how their customers feel.
Real-Time Insights
With Real-Time Sentiment Analysis with NLP for Customer Feedback, companies can immediately monitor customer attitudes. They can measure shifts in mood through instant messaging with consumers on their website, track social media mentions, or turn negative feedback into opportunities. This instant response leads to proactive customer engagement, as opposed to reactive customer service.
Enhanced Customer Experience
By recognizing repetitive pain points in negative feedback about a product or service, companies can quickly and easily address these issues using NLP Text Analytics. For example, if customers repeatedly mention a problem with a particular feature, companies can take steps to resolve the issue, thereby enhancing the overall customer experience through Advanced Sentiment Analysis with NLP.
Actionable Data
Unstructured data, such as customer reviews or social media comments, can be too vast to analyze manually. Sentiment Analysis Using NLP for Customer Feedback turns this unorganized information into usable insights. When sentiments are grouped together and trends are highlighted, teams can more easily identify areas that require attention during decision-making, basing their actions on facts rather than guesswork. This leads to better strategic decisions, improved targeting, and overall enhanced business performance.
Cost Efficiency
Manual sentiment analysis is time-consuming and resource-draining, as significant amounts of information must be processed and interpreted before a conclusion is reached. Applying NLP Algorithms for Sentiment Analysis to feedback analysis helps save time and allows businesses to focus more effort on other important areas. While customer complaints vary, Natural Language Processing Sentiment Analysis allows businesses to manage them instantly, making the process more cost-effective.
Emotion Detection
Beyond the typical classification of feedback into positive, negative, or neutral, Emotion Analysis NLP also detects additional emotions such as anger, happiness, sadness, or neutrality. This improves both product delivery and customer relations. For example, excitement can be valuable for leveraging public opinion in marketing, while early detection of customer frustration can help prevent unnecessary escalations through Sentiment Extraction Using NLP.
Improved Decision-Making
Natural Language Processing Techniques for Sentiment provide businesses with a better understanding of how customers feel about different company operations. This enables data-driven decision-making regarding service delivery. NLP Models for Text Classification empower companies to make improvements that could boost customer loyalty and retention, such as enhancing customer service protocols or developing new product features.
Use Cases of NLP Sentiment Analysis for Customer Feedback
NLP Sentiment Analysis for Customer Feedback is a versatile tool in customer feedback management that helps organizations gain a better understanding of their clients. Below are some key use cases of Sentiment Analysis Using NLP for Customer Feedback:
- Monitoring Social Media Sentiment with NLP
Companies often use NLP Sentiment Analysis for Customer Feedback to understand public perception of their brands on social media platforms. By analyzing tweets, comments, and posts, businesses can detect patterns, address customer issues, and respond proactively, improving customer loyalty when positive mentions are identified. - Improving Product and Service Offerings with NLP Models
Organizations use NLP Models for Sentiment Analysis to identify recurring problems or successful features based on customer reviews and feedback. For instance, if a product is frequently associated with poor usability, NLP Text Analytics can pinpoint areas of improvement for better customer experiences. - Enhancing Customer Support with NLP Algorithms
NLP Algorithms for Sentiment Analysis help customer service representatives prioritize their work queue based on the emotional tone of interactions. Tickets with highly negative sentiment can be escalated for quicker resolution, improving customer satisfaction and support efficiency. - Survey Analysis with NLP Text Mining
NLP for Sentiment Analysis has simplified the analysis of open-ended survey responses by grouping sentiments and identifying common themes. This process, powered by Text Mining Using NLP, allows companies to gain valuable insights without the need to manually read through every response. - Competitor Benchmarking Using NLP Sentiment Analysis
By analyzing public feedback on various brands, businesses can assess their strengths and identify areas for improvement. Sentiment Analysis Natural Language Processing makes competitor benchmarking more insightful by highlighting trends and emotions expressed across multiple platforms. - Real-Time Crisis Management with NLP Sentiment Analysis
Real-Time Sentiment Analysis with NLP for Customer Feedback enables businesses to identify instances where customers are expressing negative sentiments about a company’s products or services. This helps in intervening quickly and addressing potential issues in real-time. - Personalized Marketing with NLP Sentiment Analysis
By understanding consumer moods and emotions, Emotion Analysis NLP allows companies to tailor marketing messages that resonate with specific customer segments. Sentiment Extraction Using NLP empowers businesses to craft more personalized campaigns, improving overall customer experience.
Challenges in NLP Sentiment Analysis and How to Overcome Them
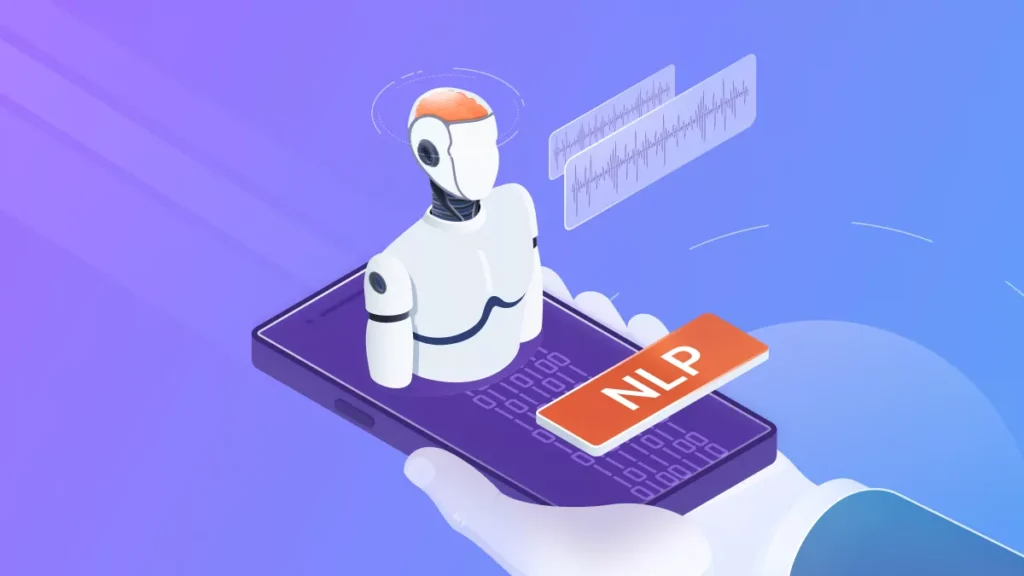
While Sentiment Analysis in NLP has some challenges, it is still a very powerful tool. Several challenges must be addressed if businesses are to realize its full potential. For example, businesses need to overcome a range of issues, from the use of contextualized language to processing disparate data sources. Some of the common challenges include the following:
Understanding Context and Nuance
Challenge: NLP struggles to properly understand context, particularly with sarcasm and ambiguous statements. For example, the statement “Great job ruining my day” might be wrongly classified as positive without understanding the context.
Solution: Use more advanced NLP Models for Sentiment Analysis, such as deep learning-based or transformer architectures like BERT, which are designed to detect and analyze contextual subtleties.
Handling Multilingual Feedback
Challenge: Customer feedback often comes in different languages, which poses a problem for NLP Sentiment Analysis for Customer Feedback systems that are specifically trained on a single language.
Solution: To ensure accurate Sentiment Analysis Using NLP for Customer Feedback across multiple languages, use multilingual NLP Models for Sentiment Analysis or language-specific training datasets.
Dealing with Domain-Specific Language
Challenge: Industry-specific jargon or slang may confuse general-purpose NLP Algorithms for Sentiment Analysis tools.
Solution: Train specialized NLP Models for Text Classification using datasets that are specific to a particular industry in order to better understand domain-specific language and sentiment.
Classifying Mixed Sentiments
Challenge: Some feedback contains both positive and negative sentiments, making it difficult to analyze. For example, “I love the product, but the shipping was terrible.”
Solution: Classify feedback based on individual sentences or phrases rather than analyzing the entire feedback as a whole, using fine-grained Emotion Detection in NLP and Sentiment Extraction Using NLP.
Data Imbalance
Challenge: Imbalanced sentiment distributions across positive, negative, or neutral categories can lead to poor model performance during training.
Solution: Implement data augmentation methods or gather more data samples to ensure the training dataset has a balanced sentiment distribution for Best Sentiment Analysis Models.
Real-Time Processing
Challenge: Processing large volumes of live feedback can strain computational resources, leading to a degradation in model performance.
Solution: Utilize lightweight architectures and scalable cloud solutions to optimize NLP Models for Sentiment Analysis for Real-Time Sentiment Analysis with NLP for Customer Feedback.
Privacy Concerns
Challenge: Analyzing customer data often involves manipulating sensitive information, raising concerns over privacy and confidentiality.
Solution: To ensure ethical practices in NLP-based Sentiment Analysis, follow GDPR guidelines and use Natural Language Processing Techniques for Sentiment that are designed to protect privacy.
How Wizr AI Is Shaping the Future of NLP Sentiment Analysis for Customer Feedback
At a time when customer-centricity defines the new normal for competition, innovative tools like Wizr AI are transforming how feedback is analyzed and acted upon. Using cutting-edge Natural Language Processing Sentiment Analysis techniques, Wizr AI enables companies to gain deeper insights into customer sentiment, setting new benchmarks for precision and effectiveness in NLP Sentiment Analysis for Customer Feedback.
Transformative Features of Wizr AI in Sentiment Analysis Using NLP
Advanced Contextual Understanding
Wizr AI stands out from traditional sentiment analysis tools because it can interpret the context of consumer feedback holistically. The platform utilizes deep learning NLP Algorithms for Sentiment Analysis that understand sarcasm, idiomatic expressions, mixed feelings, and other nuances, ensuring more accurate results every time.
Real-Time Feedback Analysis
Using Wizr AI to process customer feedback allows companies to detect mood changes and address immediate needs when necessary. This feature is particularly important during crisis periods or when customer engagement is critical. Real-Time Sentiment Analysis with NLP for Customer Feedback allows companies to act quickly and effectively.
Multilingual and Multicultural Analysis
Wizr AI supports multiple languages, ensuring that businesses operating in global markets can gain insights from any region. The tool also detects the directionality of language through cultural calibration parameters that account for linguistic variations and regional specificity, enhancing the accuracy of Text Mining Using NLP.
Customizable Sentiment Models
Whether in e-commerce, healthcare, finance, or other industries, Wizr AI’s customizable sentiment models can be tailored to any field. This means that the tool can be trained to understand how people communicate within a specific domain, enhancing comprehension and insight into NLP Models for Sentiment Analysis.
Actionable Insights Through Dashboards
Wizr AI provides decision-makers with clear patterns and trends, allowing them to prioritize actions based on the insights gathered from NLP Text Analytics. This approach helps businesses derive actionable insights from customer feedback, leading to improved decision-making.
Scalability for Big Data
With robust infrastructure capable of managing vast amounts of data from multiple sources, Wizr AI delivers accurate, real-time Sentiment Analysis Using NLP for Customer Feedback regardless of volume or speed. This scalability ensures that large corporations benefit from continuous, high-quality analysis.
Conclusion
In the contemporary world powered by data, understanding customer sentiments is more important than ever, and NLP Sentiment Analysis for Customer Feedback has turned out to be a valuable asset in unlocking customer feedback’s genuine value. Businesses can derive deeper insights, make better decisions, and enhance overall customer experience through leveraging advanced technologies like Wizr AI. Natural Language Processing Sentiment Analysis stands at a point where it will shape the future of customer feedback management due to real-time analysis, contextual understanding, and scalable solutions. This technology paves the way for endless possibilities, enabling organizations to not only listen to their customers but also understand them better, thereby building stronger relationships and growing their businesses.
FAQs
1. What is NLP sentiment analysis in customer feedback?
NLP sentiment analysis uses natural language processing to identify emotions and opinions in customer feedback. It classifies responses as positive, negative, or neutral, offering businesses actionable insights to improve service quality. Wizr AI leverages advanced NLP to analyze feedback at scale, helping organizations understand and act on customer sentiment more effectively.
2. How does NLP sentiment analysis improve customer experience?
By analyzing large volumes of customer feedback in real time, NLP sentiment analysis helps businesses detect trends, resolve pain points faster, and personalize responses. This enhances customer experience across all touchpoints. Wizr AI’s sentiment analysis capabilities enable customer-centric decision-making powered by AI insights.
3. What are the advantages of NLP neutrality in sentiment analysis?
The advantage of NLP neutrality is that it accurately captures mixed or ambiguous feedback that may not be clearly positive or negative. This allows for a more balanced and comprehensive understanding of customer opinions. Wizr AI uses NLP neutrality scoring to fine-tune sentiment insights and support more precise feedback classification.
4. How can sentiment analysis benefit industries like transportation or aviation?
Industries like transportation benefit from passenger feedback and sentiment analysis solutions by identifying service issues, optimizing staff responses, and improving customer satisfaction. Wizr AI provides customizable sentiment analysis tools that help travel and transport businesses decode passenger experiences efficiently.
5. Why should companies use NLP-based tools for feedback analysis?
NLP-based tools automate the process of extracting insights from customer comments, reducing manual effort and increasing accuracy. They help companies monitor brand perception and customer loyalty trends. Wizr AI’s feedback analysis platform combines NLP and AI to turn unstructured feedback into actionable insights that drive continuous improvement.
About Wizr AI
Wizr AI is an Advanced Enterprise AI Platform that empowers businesses to build Autonomous AI Agents, AI Assistants, and AI Workflows, enhancing enterprise productivity and customer experiences. Our CX Control Room leverages Generative AI to analyze insights, predict escalations, and optimize workflows. CX Agent Assist AI delivers Real-Time Agent Assist, boosting efficiency and resolution speed, while CX AutoSolve AI automates issue resolution with AI-Driven Customer Service Automation. Wizr Enterprise AI Platform enables seamless Enterprise AI Workflow Automation, integrating with data to build, train, and deploy AI agents, assistants, and applications securely and efficiently. It offers pre-built AI Agents for Enterprise across Sales & Marketing, Customer Support, HR, ITSM, domain-specific operations, Document Processing, and Finance.
Experience the future of enterprise productivity—request a demo of Wizr AI today.
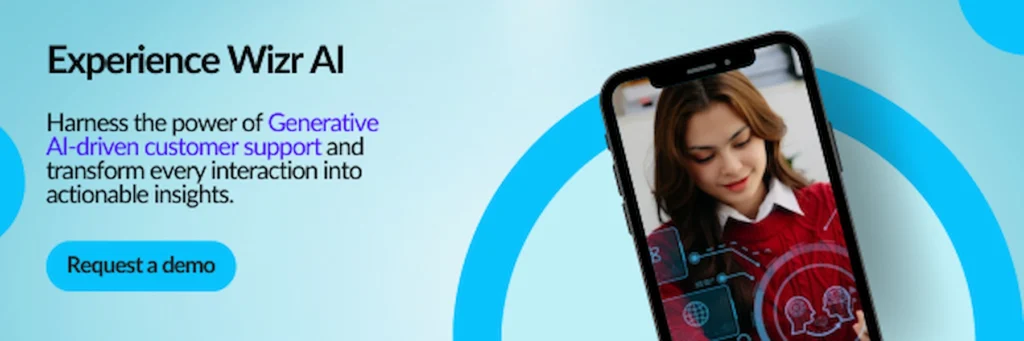