Introduction
Understanding customer needs, likes, and preferences is central for any business to thrive in this competitive world. Interpreting customer behavior from the various data revolving around purchases and interactions involves a process called Predictive Customer Analytics. Businesses today are adopting Predictive Customer Analytics Solutions and prioritizing this process to both grow and expand. Companies use the insights extracted from these data to strategize new products and services, deliver wholesome experiences, and build a highly profitable business.
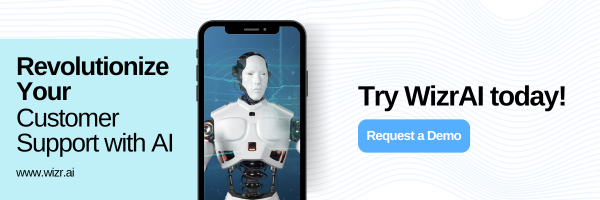
Customer analytics goes way back in history when, in the 80s, companies began to look deeper into their products, and creative ideas emerged to popularize their products amongst others in the market. This involved peeking into the lives of the customers through surveys and feedback forms to collect data. Today, with the evolution of digital interactions and social media, the touch points from where companies can collect their data are vast. The reach into customers’ wants and likes has become faster and more precise, making it easy for businesses to make informed decisions, predict customer behavior, and utilize these in their operations to maximize customer satisfaction, loyalty, and revenue.
The global Predictive Analytics for Customer Insights market was valued at USD 18.89 billion in 2024 and is projected to grow at a CAGR of 28.3% from 2025 to 2030. (Source)
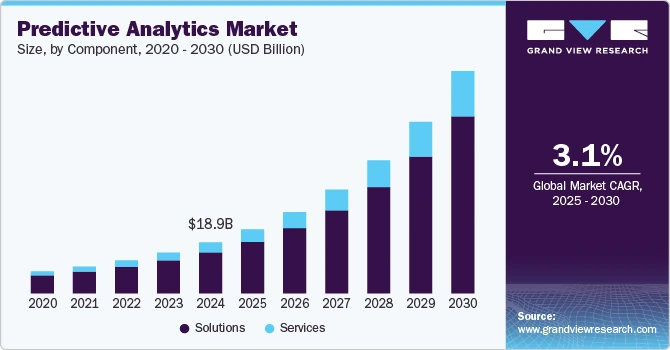
What is Predictive Customer Analytics?
Predictive Customer Analytics Platforms are AI-powered, machine learning-enabled platforms that use data to interpret the patterns and sentiments in them. The learning data interpreted is then used to factually predict an outcome that assists organizations in creating good experiences for their customers, implementing better processes in their operations, or delivering services that are meaningful to the community.
Why do we need Predictive Analytics?
According to the Zendesk Customer Experience Trends Report 2023, 67 percent of business leaders report seeing disorganized efforts using and sharing customer data. Despite having access to answers regarding Predictive Analytics for Customer Behavior, many companies are still relying on guesswork. (Source)
In this digital era, customers are educated about products and services and have all the information at their fingertips to make intelligent decisions. They have various options that would enable them to browse and decide on the right product that fits perfectly with what they want. This makes it crucial for every company to be always aware of what customers are looking for. In a more promising scenario, if organizations can predict what products or services the customer would need next by understanding their current activities and interactions, it would be very lucrative for their growth. This would enhance the opportunities for promotions and campaigns to their audience, enabling them to make inspired purchases.
As glorious as this is for the business, it would require an AI Predictive Customer Analytics Tool with access to a detailed and clear customer database to make accurate predictions. Without this precision, the analysis remains speculative and not factful.
How does Predictive Analytics work?
A Predictive Customer Analytics Platform would delve into the organization’s customer base and create a singular view that enhances the predictability of sustaining existing customers and their interests, acquiring new customers, and retaining them. It would also aid in identifying high-net-worth customers and recommend methods of continued interaction, creating opportunities for the company. AI-powered Predictive Customer Analytics will enable the following:
- Forecast customer behavior expected, which would set market trends for businesses.
- Use historical data and build models that predict customer movement, saleability, and trends in industries.
- Automate the analytics process and provide fast and instant results that are actionable.
- Personalized marketing, which would enhance the customer experience and lead to revenue generation.
- Accurate predictions through continuous monitoring in compliance with quality data.
These predictions are crucial in an evolving and constantly fluctuating market. Predictive Analytics for Business would significantly elevate the business, enabling it to adapt to a highly competitive economy. Wizr Customer Intelligence Hub uses Generative AI to turn customer support interactions into valuable insights. A well-built AI-powered Predictive Customer Analytics Platform ensures data analysis through automation and has advantages such as:
- Quick assimilation of vast and complex data
- Get analyzed data frequently
- Results that may lead to relevant and beneficial actions
- Collecting personalized details on customer experiences
- Efficient and accurate results with improved machine learning for Predictive Customer Analytics
- Reduced costs on customer research and data collection
Top 8 Predictive Customer Analytics Trends to Watch in 2025
In the last decade, technological advancements have geared customer interactions across industries. With the introduction of AI Predictive Customer Analytics, businesses began interacting with customers on a personalized level, following their internet usage, extracting information from their browsing history, curating experiences from the information collected, and offering it to them like a well-wisher. However, these are now the run-of-the-mill norms, and in 2025 there will be a need for a step up. Organizations will look forward to Predictive Customer Analytics AI displaying next-level intelligence and practices. Some of the Predictive Customer Analytics Trends that would shape how businesses operate in 2025 and the near future are:
1. AI and Machine Learning in Predictive Customer Analytics
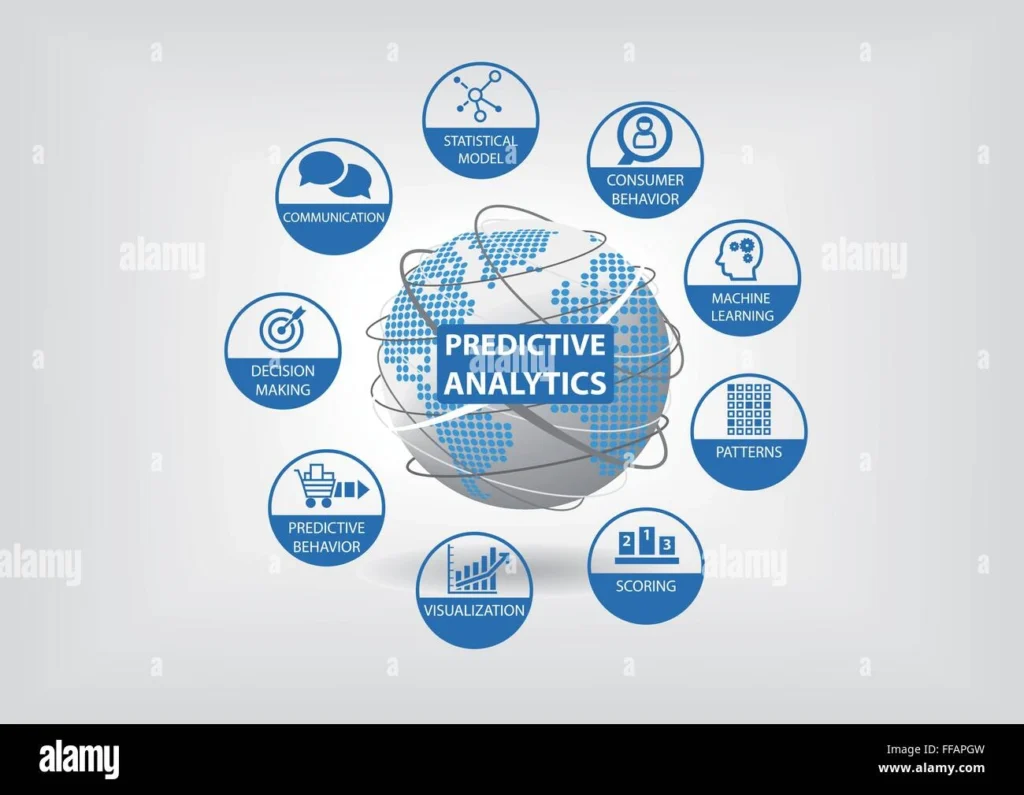
Predictive Customer Analytics Tools powered by Machine Learning for Predictive Customer Analytics have AI at the core of their operating processes. Predictive Customer Analytics Algorithms are trained to recognize data patterns and relationships from users’ browsing and interaction history. This training further prepares the ML algorithms to identify similar unseen patterns to predict future outcomes. Estimates based on insights precipitated from continuously gathered data give a clear and factful prediction. ML algorithms and models thrive on vast amounts of data that are fed into them, and continuous training on new data produces multi-dimensional results.
Enabling Natural Language Processing (NLP) and Natural Language Understanding (NLU) in machine learning is a trend that would ensure that data inputted in simple language is inferred well to deliver clear insights. Another interesting technology that is in development and can create potential Predictive Customer Analytics Trends in complex industries such as engineering and medicine is ML’s ability to use neural networks and engage in deep learning to produce fine and realistic Predictive Analytics for Customer Insights.
AI Predictive Customer Analytics does not work singularly but with various components to infer and provide insights that are accurate and actionable. The components that work with AI are Data, Algorithms, and Predictions. These systematically work together in a series of tasks to transform raw data into highly valuable predictive insights. The various tasks and some of the Predictive Customer Analytics Tools used to perform these tasks are:
- Data Collection and Preparation with Predictive Customer Analytics Tools
- Sources: CRM systems, social media, website analytics, surveys.
- Tools: Google Analytics, HubSpot, Salesforce.
- Model Building and Training for Predictive Analytics for Customer Behavior
- Methods: Machine learning algorithms like linear regression, and decision trees.
- Tools: TensorFlow, Scikit-learn, R.
- Validation and Testing in Predictive Customer Analytics Solutions
- Deployment and Real-time Predictions
- Techniques: Pattern identification, trend analysis, correlation studies.
- Tools: Excel, Python, SAS, SPSS.
- Continuous modelling and Improvement
- Methods: Machine learning algorithms like linear regression, and decision trees.
- Tools: TensorFlow, Scikit-learn, R.
2. Real-Time Predictive Customer Analytics for Instant Insights
Live data can be used to extract predictions about events immediately, ensuring that organizations can take quick actions and decisions. This ability of Real-Time Predictive Customer Analytics can be employed across various industries to avert situations or avoid endangerment or crises. This is particularly useful in Predictive Analytics for Customer Insights in security to detect illegal activities, Predictive Analytics for Customer Behavior in banking for fraud transactions, or in engineering to send warning signals for equipment failure. Some industries that may largely benefit from Predictive Analytics for Business in real-time are:
- Insurance Sector: Insurance companies can benefit from Predictive Customer Analytics Solutions, where they can curate competitive policies for their customers while keeping risk assessment in mind on the spot.
- Financial Services: Financial services can use Predictive Customer Analytics Tools to predict probabilities of loan defaulters and decide whether to proceed with their application by providing insights into credit history and current market conditions.
- Retail & Consumer Goods: Retailers and consumer goods companies can utilize Best Predictive Customer Analytics Software to gain insights and build better customer relations. The insights into Predictive Analytics for Customer Behavior and purchase patterns can be used to create campaigns, forecast future sales, or make suggestive sales while the customer is browsing.
- Healthcare & Medical: Healthcare and medical companies can delve into historical data, demographic patterns, and lifestyle insights to analyze outcomes and provide more cost-effective, responsive, and targeted services. Predictive Analytics for Customer Retention enables the implementation of personalized care plans that can eliminate causes for readmission.
- Energy & Utilities: Energy and utilities can ensure safer operations and reduce risks by using Predictive Customer Analytics AI. Establishments use Predictive Customer Analytics Algorithms and previous data to determine maintenance schedules and preventive repairs. This approach proactively creates a safer work environment. Additionally, Predictive Customer Analytics Use Cases show how energy requirements can be understood using data from past consumption patterns and other influencing factors, allowing for better resource allocation.
- Manufacturing & Supply Chains: Manufacturing and supply chains can use Advanced Predictive Customer Analytics to empower operations to work with precision, thus enhancing customer satisfaction effectively. By analyzing factors like market trends, seasonality, and Predictive Analytics for Customer Insights, companies can accurately forecast demand and maintain optimum inventory, helping reduce resource costs.
- Public Sector: The public sector may plan projects in alignment with the needs of the community. Predictive Customer Analytics Case Study Examples demonstrate how analyzing demographics, growth patterns, and migration trends leads to better management of public works and investments for improved infrastructure.
3. Predictive Analytics for Customer Retention and Churn Prevention
Predictive customer analytics works with customers’ data to analyze their past behavior and predict what to expect in the future. This offers an opportunity to react to Predictive Customer Analytics Trends and market shifts quickly, staying ahead of the competition. Predictive customer analytics dives deep into historical data and extracts specific behaviors and traits. This enables the business to learn the customer’s journey before a purchase is made. Companies can utilize these insights to make the journey easier, offer instant options, or even curate an experience based on history. Some of the specific benefits of Predictive Analytics for Customer Retention are:
- Enhancing Customer Experience: By navigating the customer as per his or her preferences, businesses can enhance the customer experience using AI Predictive Customer Analytics. Personalized suggestions, specified product promotions, and targeted advertising can bring overall satisfaction for the customer, ultimately enhancing the experience. Proactive solutions and pre-empting customer concerns are integral to Predictive Analytics for Customer Behavior.
- Boosting Sales and Revenue: Predictive business analytics is a boon for any business. Identifying potential customers, following up on leads, strategizing pricing experiences, and curating specialized Predictive Customer Analytics Marketing campaigns to target specific market segments are all benefits of Predictive Customer Analytics Tools. These can help increase sales and revenue. Forecasting near-future demands is another advantage of Predictive Analytics for Business that can help companies optimize their operations and resources.
- Reducing Customer Churn: Reducing customer churn is essential for any business. With Predictive Analytics for Customer Churn, it is possible to identify indicators that may point towards a disgruntled customer or one who has not returned. This information aids the company to then proactively interact with the customer and create personalized experiences that may help retain them and reduce churn rates. Continuous analysis of customer feedback ensures that companies can track customer sentiments and keep improving products and services.
4. Advanced Predictive Customer Analytics for Personalization
Businesses using advanced Predictive Customer Analytics and personalization techniques see a 20% increase in revenue, while 91% of consumers are more likely to shop with brands that provide relevant offers and recommendations. Today, companies leveraging AI Predictive Customer Analytics report 60% higher customer satisfaction rates. (Source)
AI and Predictive Customer Analytics Solutions are key drivers in implementing personalization in customer experience and strategizing loyalty. To employ precision in personalization, the AI must be trained on high-quality and accurate data. While AI enables micro-segmentation insights for hyper-personalized experiences, the matter of data privacy must also be considered and respected. In the present scenario, brands are creating convincing personalized experiences for customers using AI Predictive Customer Analytics to:
- Leverage advanced handling of data.
- Generate multi-dimensional content and insights.
- Micro-segment the target market and customer.
- The Role of Data in Predictive Customer Analytics
To ensure absolute personalization, the factors that play the prime role in Predictive Analytics for Customer Insights are the data.
Data forms the spine of personalization. The larger the database, the more accurate the insights derived. When AI Predictive Customer Analytics and data analytics work together, data can be gathered from numerous sources of customer interaction across the web. This allows Predictive Analytics for Customer Behavior to browse through vast amounts of information and obtain a very deep understanding of the customer’s behaviors and interests.
The data is scanned, and Machine Learning for Predictive Customer Analytics enables insights into trends and forecasts outcomes. This further opens up the possibility of personalization for the customer. With micro-segmentation by AI, brands are going deeper and creating niche groups within segments to extract varying needs and present possibilities to influence buyer behavior.
Going beyond Predictive Analytics for Customer Retention, Prescriptive Analytics allows companies to engage in even more personalized conversations with customers and gather direct data to make recommendations. A key ability here is to adapt instantly to the change in need in Real-Time Predictive Customer Analytics, contributing to a very satisfying shopping experience for the customer.
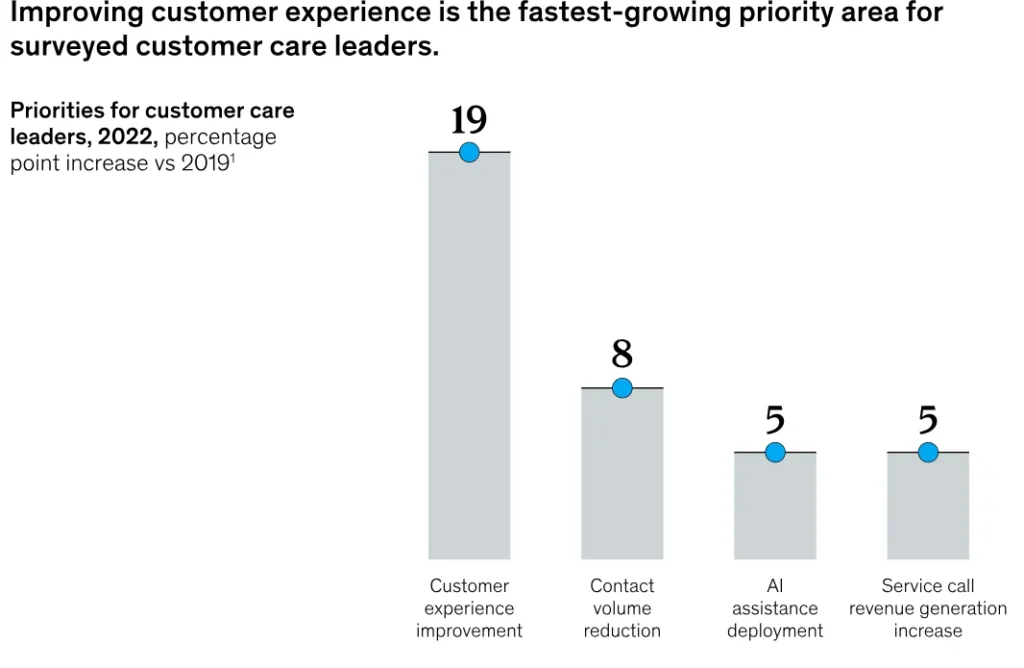
5. Predictive Customer Analytics for Optimizing Marketing Strategies
Through Predictive Analytics for Customer Churn, once insights into customer preferences, demographics, and shifts in interests are gathered, a customized marketing campaign will target and influence customers segment-wise. This would enable optimum strategies to be employed in marketing products and services specific to customer groups.
The ability to approach different groups with specific products, promotions, and advertising greatly enhances the organization’s revenue-generating capabilities, leading to the growth of business through Predictive Customer Analytics Marketing.
6. Predictive Customer Analytics for Contact and Call Centers
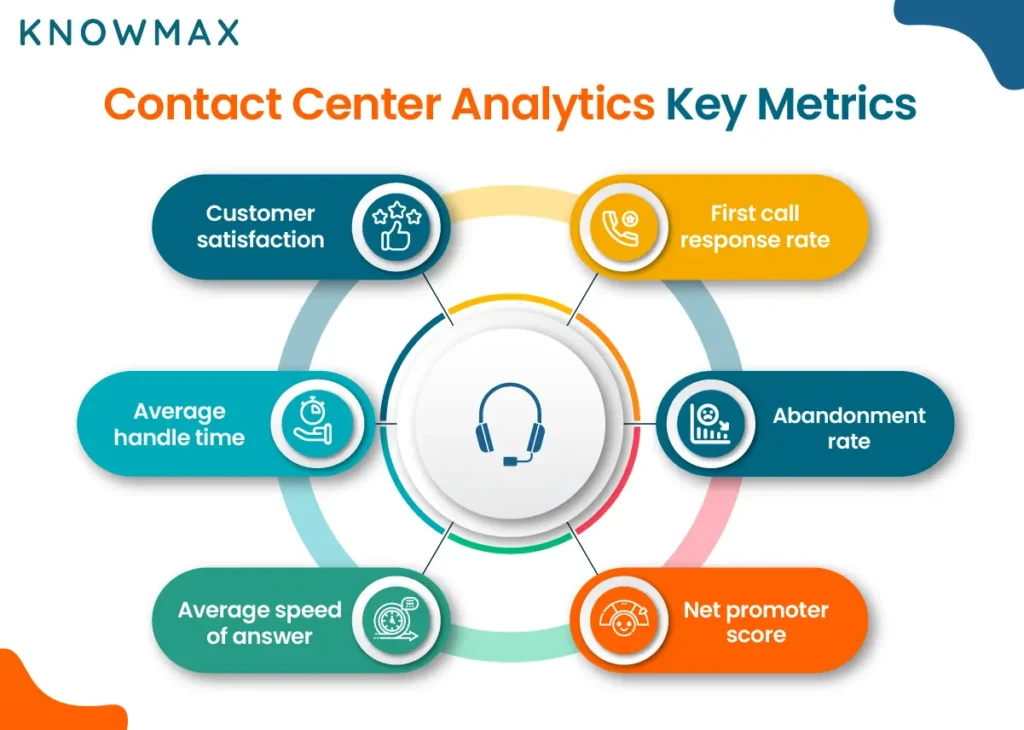
Any contact or call center is traditionally cued to answer queries or solve issues. The support agents aim towards quick responses and first-call resolution to provide efficient customer service. Applying Predictive Customer Analytics in this area would bring proactiveness and elevate customer service to the next level. Using historical data and statistics, AI Predictive Customer Analytics can pre-empt issues and forecast the needs of customers, reducing the need for them to call. Agents can forward solve issues by initiating communication or cautionary advice on issues that lead to frequent calls. Also, when a customer calls, Predictive Analytics for Customer Insights gives insights into which customer service agent last solved the customer’s issue and can route it there for continuity and amicability.
The use of Predictive Customer Analytics Tools in contact centers has led to many uses. Some of the well-known ones are:
- Predicting hold time for customers waiting on the phone. This helps in giving clarity to the customer and managing the queue.
- Predicting call volume and peak call times enables call center managers to schedule rosters and route calls to meet demand. The AI uses historical data to predict changes in call volume over time and provide insights into peak and low traffic hours.
- Routing calls to the best available agent is possible through Predictive Customer Analytics Solutions. It uses customer data to understand the needs of the customer and the agents best-suited to handle them.
- Conversation intelligence software uses Predictive Analytics for Customer Behavior to interpret the language of the customer and agent and offer insights that can help make informed decisions.
7. Predictive Customer Analytics Solutions for Improving CX
The evolution of Predictive Customer Analytics was first used to build customer experience. The impact of AI Predictive Customer Analytics on the way companies manage this experience is exciting. Insights from Predictive Analytics for Business have helped companies foster connections with customers and reach differentiation in the highly competitive market today. Customer experience has reached heights using Predictive Analytics in Customer Experience (CX), and some of these are:
- Build customer loyalty and satisfaction.
- Create customer value.
- Reduce customer attrition.
- Upgrade cross-selling and up-selling.
- Accelerate operational efficiency.
8. Future Use Cases and Case Studies in Predictive Customer Analytics
In the future, Predictive Customer Analytics will become an essential feature in several sectors, enhancing both business strategies and customer experiences.
1. Retail
Retailers are the leading users of Predictive Analytics for Customer Behavior, with their continuous monitoring of customer behavior to drive sales. Using Predictive Customer Analytics Tools allows businesses to forecast demand trends and optimize inventory, leading to better customer service and increased sales.
2. Healthcare
Predictive Analytics for Business is revolutionizing the healthcare sector, with institutions globally adopting AI Predictive Customer Analytics to understand the prevailing and expected health of communities. Predictive Analytics for Customer Insights can provide key insights into diseases, predict patient needs, and suggest preventive care, improving overall healthcare outcomes.
3. Sports
Professional sporting activities depend on Predictive Customer Analytics Solutions to gauge the performance of probable players and help managers and coaches assemble the best teams for a game. Machine Learning for Predictive Customer Analytics also aids in off-field assessments, such as evaluating the revenue a player can generate through fan engagement.
4. Weather
Accurate weather forecasts through Predictive Analytics for Customer Retention can be beneficial for various industries. By using data collected from satellites and past weather patterns, Real-Time Predictive Customer Analytics can provide precise forecasts that help industries make informed decisions and plan accordingly.
5. Human Resources
In human resources, analyzing data related to personnel can help predict promotions, appraisals, and increments. Predictive Analytics for Customer Churn can also predict the likelihood of employee retention, while models built on vast data of working hours, shifts, and reviews can provide valuable insights for workforce planning and talent acquisition.
Some of the case studies in successful Predictive analytics are
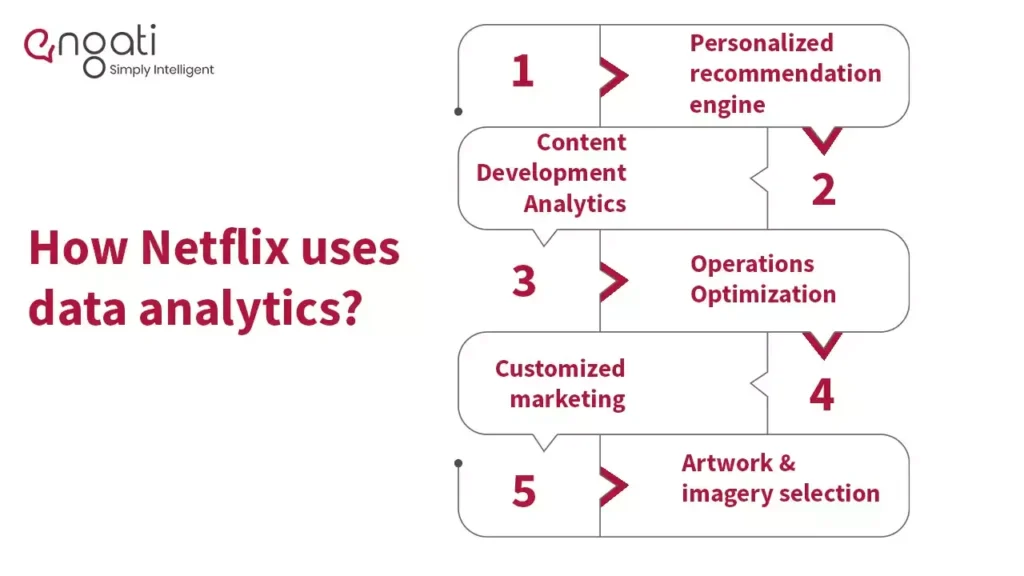
Some of the most successful implementations of Predictive Customer Analytics AI are seen in the following case studies, where predictive models have transformed customer interactions and business outcomes:
- Hopper: A mobile app that uses historical data and Machine Learning for Predictive Customer Analytics to predict flight prices. It provides users with recommendations for the best dates to book flights, offering better prices based on Predictive Analytics for Customer Insights.
- Netflix: Uses Predictive Customer Analytics Algorithms to analyze viewing preferences, personalize recommendations, and curate content that keeps users engaged. This personalization strategy enhances the user experience and improves retention.
- Spotify: Leverages Predictive Analytics for Business to create playlists, suggest artists, and elevate the overall music experience. By using data-driven insights, Spotify tailors its recommendations to user tastes, keeping them engaged with new content.
- Amazon: Amazon’s recommendation engine is powered by Advanced Predictive Customer Analytics, using Predictive Customer Analytics Use Cases to drive sales through suggestive selling of relevant products based on historical customer behavior. This boosts conversion rates and increases revenue through personalized recommendations.
Conclusion: The Future of Predictive Customer Analytics in 2025
Amazon reported a remarkable 35% increase in customer retention in 2024, showcasing the significant impact of advanced data strategies. (Source)
In 2025, Predictive Customer Analytics will become the keystone requirement for businesses aiming to leverage customer experience in this highly competitive world. The need to understand customers and continuously innovate to capture their attention will outshine all other key areas of business. Predictive Analytics for Customer Insights will not just be about compiling and sorting large amounts of data but will use AI Predictive Customer Analytics to gain augmented insights and ensure high-end data protection. The trends in the coming years will enable organizations to elevate customer experiences and streamline processes for efficiency, leading to more informed decision-making.
If 2024 was about AI and personalization, 2025 will open doors for critically evolved AI Predictive Customer Analytics Tools geared toward Predictive Analytics for Customer Behavior. Shifts in market demands will be anticipated well in advance, leading to better strategies and sustainable growth.
FAQs
1. What is Predictive Customer Analytics and how does it impact customer support?
Predictive Customer Analytics uses data-driven insights to forecast customer behavior, preferences, and needs. By leveraging AI Predictive Customer Analytics, businesses can proactively address customer queries, predict future trends, and enhance customer satisfaction. This leads to more efficient customer support, improved retention, and reduced churn. Predictive Analytics for Customer Insights plays a crucial role in anticipating issues before they arise, helping support teams deliver a more personalized experience.
2. How does AI Predictive Customer Analytics differ from traditional analytics?
Unlike traditional analytics, which primarily focuses on historical data, AI Predictive Customer Analytics uses advanced machine learning algorithms to identify patterns and predict future customer behavior. This enables businesses to forecast demand, enhance customer service, and optimize processes, creating a more personalized and efficient customer experience. Machine Learning for Predictive Customer Analytics offers businesses the ability to make real-time, data-driven decisions that are key for competitive advantage.
3. What are the key benefits of using Predictive Analytics for Customer Retention?
Predictive Analytics for Customer Retention helps businesses understand customer behavior, predict churn, and develop targeted strategies to enhance loyalty. By utilizing Predictive Customer Analytics AI, companies can identify at-risk customers early and engage them with personalized offers, reducing churn rates and improving long-term customer relationships. This allows for data-driven decisions that increase retention and satisfaction rates.
4. What tools are available for Predictive Customer Analytics in customer support?
Several Predictive Customer Analytics Tools can help businesses improve their customer support strategies. These tools use AI Predictive Customer Analytics to provide actionable insights for customer behavior, predict customer needs, and optimize support team performance. Real-Time Predictive Customer Analytics tools can help businesses respond faster, improve service quality, and ensure a more personalized customer experience.
5. Can Predictive Customer Analytics be used to improve customer experience in contact centers?
Yes, Predictive Analytics for Customer Insights in contact centers is a game-changer for improving customer experience. By utilizing Contact Center Predictive Customer Analytics, businesses can predict call volumes, route customers to the best agents, and forecast hold times, ensuring a smoother and more efficient customer interaction. Real-Time Predictive Customer Analytics further enhances decision-making in contact centers, helping improve overall customer satisfaction.
About Wizr AI
Wizr enhances customer support seamlessly with AI-powered customer service tools. Cx Hub predicts and prevents escalations, while Agent Assist boosts productivity with automated customer service software. Auto Solve handles up to 45% of tickets, freeing agents for complex issues. Cx Control Room analyzes sentiment to guide proactive solutions, maximizing satisfaction and retention. Guided by generative AI for customer support, Wizr prioritizes exceptional customer experiences. To explore how these tools can benefit your business, request a demo of Wizr AI today.
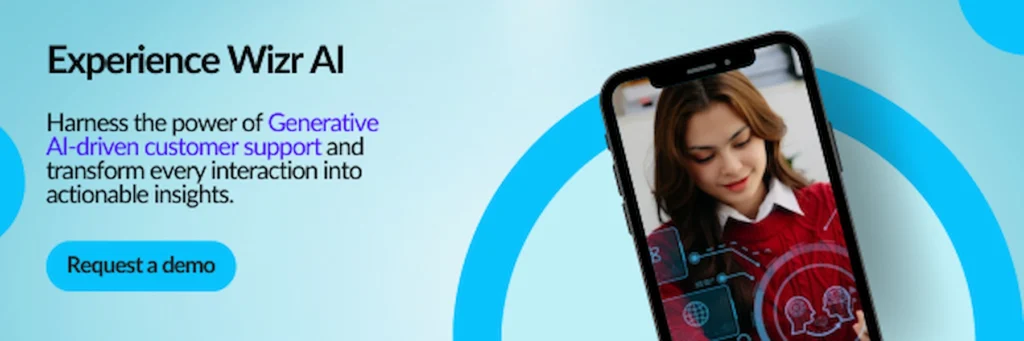
NLP vs NLU vs NLG: How Chatbot AI Improves Customer
AI-driven chatbots are transforming customer service because they enable efficient, responsive, and human-like interactions. Nonetheless, this power is based on…
8 Predictive Customer Analytics Trends to Watch in 2025
Introduction Understanding customer needs, likes, and preferences is central for any business to thrive in this competitive world. Interpreting customer behavior…
AI-Powered SaaS Customer Support: 10 Best Practices for 2025
As technology advances, AI-powered SaaS customer support is increasingly being utilized within the context of B2B AI-powered SaaS customer support…
7 Proven Ways AI Ticket Tags Can Improve Customer Service
Introduction Today, customer support agents have varied methods to address issues. AI Ticket Tags for Customer Service enhance this process as…
AI Workflow Builder: Transforming Business Automation in 2025
In 2025, businesses are in the process of changing their operational methods by leveraging the potential that AI Workflow Builders…
Voicebot vs Chatbot: Which AI is Best for Your Customer
Introduction Customer support is undergoing a revolution with the rise of artificial intelligence (AI). A recent study revealed that 67% of…