In today’s digital age, understanding and managing customer sentiment has become crucial for businesses looking to leverage the power of data-driven decision-making. Traditional methods of sentiment analysis can often fall short of capturing the nuanced and complex emotions of customers.
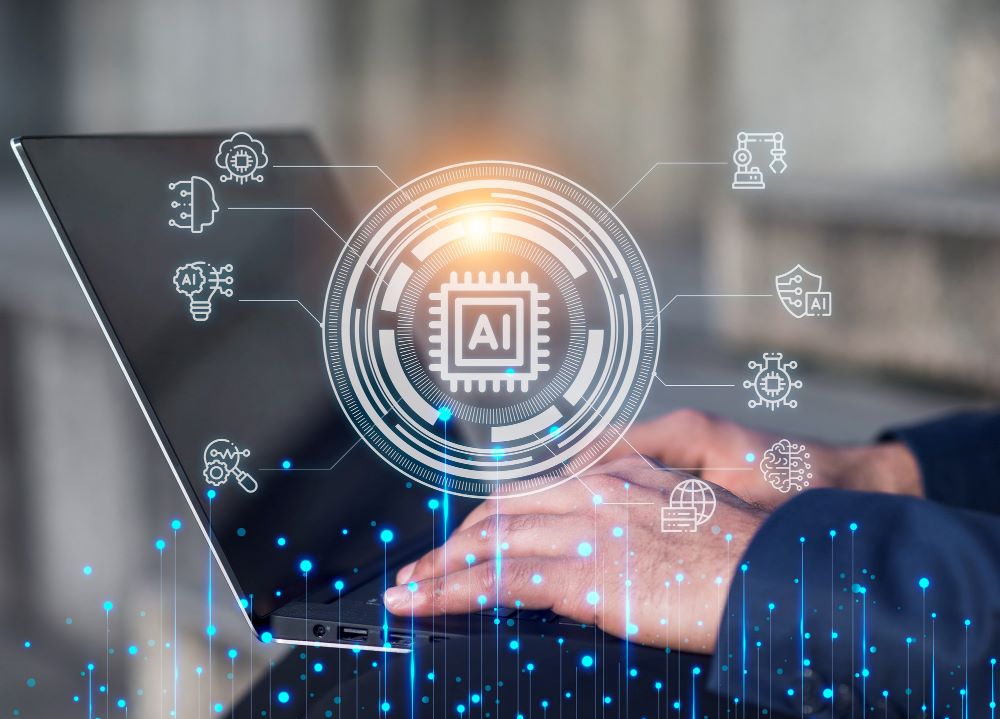
However, recent advancements in generative AI customer sentiment analysis offer exciting potential to elevate customer sentiment analysis to new heights. By integrating generative AI text analysis into existing systems, businesses can gain deeper insights into customer feedback, identify latent emotions, and ultimately enhance their ability to cater to the evolving needs and preferences of their customer base.
In this blog post, we will explore the significance of building intelligent systems by integrating generative AI for customer service sentiment analysis, the benefits of customer sentiment analysis, and practical ways businesses can harness this cutting-edge technology to drive customer-centric growth.
What is Generative AI?
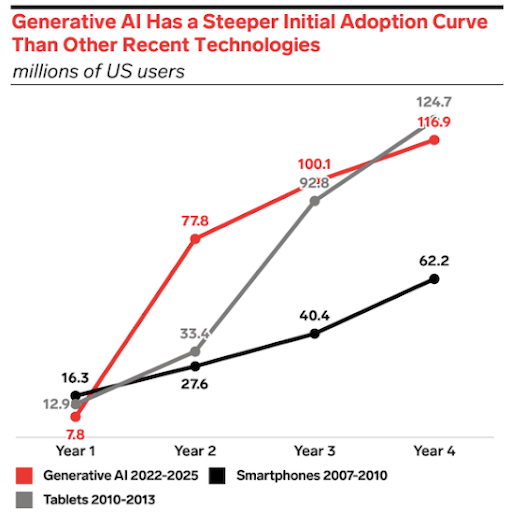
Grasping the Potential: How Generative AI Transforms Text
Generative AI customer sentiment analysis represents a groundbreaking leap in the realm of customer sentiment analysis. Unlike traditional systems that rely on predefined patterns, generative AI possesses the remarkable ability to create text that mirrors human-like expression. This transformative capability stems from its capacity to analyze vast datasets, understand context, and generate content that seamlessly fits within linguistic norms.
As businesses delve into the possibilities of generative AI for customer sentiment analysis, they open doors to more sophisticated communication strategies. Whether crafting marketing messages, generating product descriptions, or enhancing customer interactions, the potential impact on textual communication is profound.
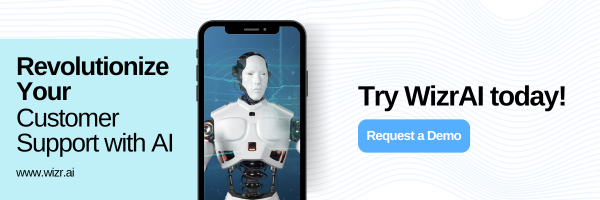
Applying Generative AI: A Key Element in Customer Sentiment Understanding
The application of generative AI for customer service sentiment analysis is a strategic move towards a more nuanced and comprehensive approach. By analyzing vast amounts of textual data, generative AI customer sentiment analysis can discern subtle shifts in sentiment, providing businesses with real-time insights into customer preferences and opinions. Its adaptive nature allows it to evolve alongside changing language trends, ensuring accurate sentiment analysis even in dynamic environments.
In practical terms, applying generative AI customer sentiment analysis means gaining a deeper understanding of customer feedback, reviews, and interactions. This invaluable tool not only enhances the accuracy of sentiment analysis but also enables businesses to proactively address concerns and capitalize on positive feedback, ultimately delivering the benefits of customer sentiment analysis that drive customer-centric growth.
Challenges of Using Generative AI for Customer Sentiment Analysis
Customer sentiment analysis plays a pivotal role in understanding and responding to customer feedback, but traditional approaches often encounter significant limitations. In this section, we delve into the challenges faced in customer sentiment analysis and explore effective solutions, with a focus on leveraging generative AI for customer service sentiment analysis.
To effectively enhance your understanding of this topic, it’s essential to consider best practices for customer feedback analysis. By adopting these practices, businesses can better navigate the complexities of customer sentiment and improve their overall service quality.
1. Identifying Limitations: Overcoming Hurdles in Traditional Approaches
Traditional sentiment analysis methods frequently grapple with the nuances and complexities of human language. The rigidity of rule-based systems and the struggle to interpret sarcasm, slang, or context-specific expressions pose substantial hurdles. Identifying these limitations is the first step toward enhancing the effectiveness of customer sentiment analysis tools.
To overcome these challenges, businesses need to adopt a more flexible and adaptive approach. Machine learning models, trained on vast datasets that encompass diverse language patterns, can better capture the subtleties of sentiment. By acknowledging and addressing the limitations of traditional methods, organizations can pave the way for more accurate and insightful generative AI customer sentiment analysis.
2. Moving Beyond Constraints: Solutions for More Effective Sentiment Analysis
One of the primary constraints in traditional customer sentiment analysis is the binary classification of sentiments as positive or negative. Human emotions are inherently complex and often fall within a spectrum. Moving beyond these constraints involves adopting more nuanced customer sentiment analysis techniques that account for a broader range of emotions.
Natural Language Processing (NLP) techniques in generative ai, including sentiment analysis chatgpt and sentiment intensity analysis, offer a more granular understanding of sentiment by assigning varying degrees of positivity or negativity. Sentiment analysis models that incorporate machine learning algorithms can adapt to evolving language trends, providing a more realistic representation of customer sentiment analysis using generative AI.
3. Leveraging Generative AI: A Strategic Response to Analytical Challenges
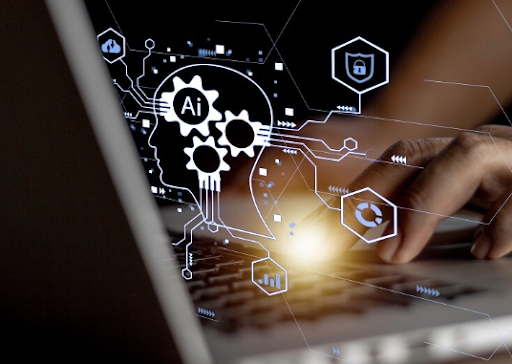
Generative AI customer sentiment analysis emerges as a strategic response to the analytical challenges inherent in customer sentiment analysis. By harnessing the power of generative AI text analysis, businesses can address the limitations of rule-based and binary classification systems. Generative AI Chatbot models, such as language models powered by deep learning, excel at generating human-like text and understanding context.
These models, trained on vast amounts of diverse textual data, can comprehend and generate language with a level of sophistication that traditional methods struggle to achieve. Leveraging generative AI in sentiment analysis enables organizations to capture the richness of customer expressions and evolving language patterns.
Benefits of Integrating Generative AI in Customer Sentiment Analysis
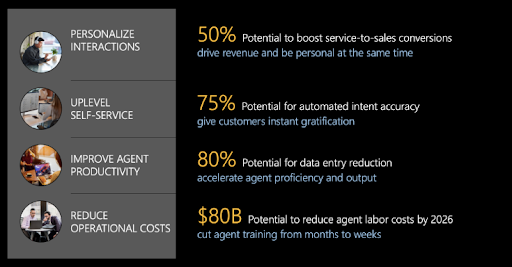
In the realm of customer sentiment analysis, integrating generative AI emerges as a game-changer, offering advantages that significantly elevate the understanding and responsiveness of customer sentiment analysis tools.
1. Enhancing Precision: How Generative AI Boosts Sentiment Analysis Accuracy
Customer sentiment is often nuanced and context-dependent, posing a challenge for traditional customer sentiment analysis tools. Generative AI steps in to address this complexity by enhancing precision and accuracy in customer sentiment analysis.
Generative AI models, equipped with advanced algorithms, excel at recognizing subtle changes and contextual cues within text data. This enables them to discern between similar expressions with distinct sentiments, ultimately refining the accuracy of sentiment classifications. The result is a sentiment analysis tool that not only captures the sentiment expressed by customers but does so with a level of precision that surpasses conventional methods.
By harnessing the power of generative AI customer sentiment analysis, businesses can elevate the reliability of their customer sentiment analysis processes, ensuring more accurate insights into customer attitudes and opinions.
2. Unveiling Nuances: A Clearer Understanding of Language with Generative AI
Language is inherently intricate, often characterized by subtle expressions and varying tones that convey multiple meanings. Traditional customer sentiment analysis tools may struggle to grasp these intricacies, leading to misinterpretations of customer sentiments.
Generative AI customer sentiment analysis, with its ability to comprehend and generate human-like text, excels at unraveling these linguistic variations. It goes beyond the surface-level analysis, delving into the intricacies of language to provide a clearer understanding of customer sentiments.
This higher-level understanding is crucial for businesses aiming to derive accurate insights from customer sentiment analysis using gen ai, enabling them to make informed decisions and enhance their overall customer experience.
3. Adapting in Real-Time: The Dynamic Advantage of Generative AI in Sentiment Analysis
Customer sentiments are dynamic and subject to change based on various factors, such as market trends, product launches, or external events. Traditional customer sentiment analysis tools, often static in nature, may struggle to adapt swiftly to these fluctuations.
Generative AI customer sentiment analysis introduces a dynamic advantage by enabling real-time adaptation in customer sentiment analysis using generative AI. Its ability to learn and evolve from incoming data allows it to stay attuned to changing customer sentiments, ensuring that businesses don’t miss out on important data.
This dynamic adaptation empowers businesses to respond promptly to shifts in customer sentiment, enabling them to proactively address concerns, capitalize on positive feedback, and stay agile in a rapidly evolving market. The real-time capabilities of generative AI redefine the landscape of customer sentiment analysis, providing businesses with a competitive edge in staying connected to the pulse of their customer base.
Use Cases of Generative AI in Customer Sentiment Analysis
Let’s explore how customer sentiment analysis using generative AI is making a tangible impact:
1. Harnessing Social Media Insights: Practical Applications of Generative AI
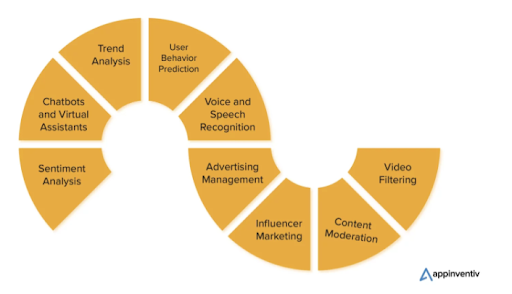
In the realm of social media, where opinions are abundant and dynamic, Generative AI customer sentiment analysis proves invaluable. By analyzing vast amounts of social data, this technology can decipher sentiments expressed on platforms like Twitter, Facebook, LinkedIn, and Instagram.
It goes beyond basic sentiment classification, delving into the subtleties of language to gauge the true sentiment behind customer posts. For businesses, this means a deeper understanding of customer opinions, enabling them to respond proactively to trends, issues, and opportunities in real-time.
Example: A retail brand uses Generative AI to analyze social media chatter surrounding a recent product launch. By capturing not just positive or negative sentiments but also the underlying emotions, the brand adapts its marketing strategy swiftly, addressing concerns and amplifying positive feedback. This highlights the benefits of customer sentiment analysis with Generative AI and underscores the impact of positive customer sentiment on brand loyalty.
2. Analyzing Reviews with Precision: Real-world Examples of Generative AI Impact
Generative AI customer sentiment analysis excels in the analysis of product reviews, providing businesses with a detailed understanding of customer feedback. Traditional sentiment analysis chatgpt might categorize a review as positive or negative, but Generative AI text analysis goes further, identifying specific aspects that customers highlight. It pinpoints key phrases, sentiments, and context, allowing businesses to pinpoint strengths and weaknesses with unparalleled precision.
Example: An e-commerce platform integrates Generative AI to analyze product reviews. Instead of just knowing if a review is positive or negative, the platform identifies specific product features or services mentioned most frequently in customer feedback. This insight guides the company in product improvements and strategic decision-making, showcasing the benefits of customer sentiment analysis using generative AI.
3. Revolutionizing Customer Support: Implementing Generative AI in Interaction Analysis
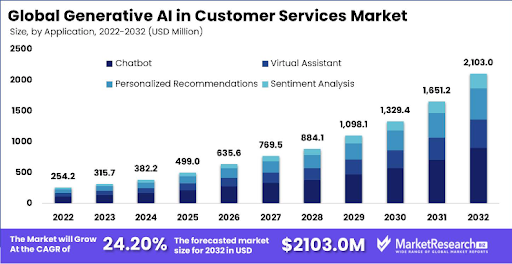
Generative AI for customer service sentiment analysis is reshaping the landscape of customer support by analyzing interactions between customers and support agents. It not only categorizes sentiments expressed by customers, but also understands the context and sentiment shifts throughout the conversation. This enables businesses to provide more personalized and effective support, identifying problems, and ensuring a smoother customer experience.
Example: A telecommunications company integrates Generative AI into its customer support system. By analyzing customer interactions, the system can identify frustration levels, common issues, and successful resolutions. This data helps the company optimize its support processes, train agents effectively, and enhance overall customer satisfaction, demonstrating the benefits of customer sentiment analysis using generative AI.
Implementing Generative AI Strategies in Customer Sentiment Analysis
Effective integration of Generative AI customer sentiment analysis requires strategic planning and thoughtful execution. Here are three actionable implementation strategies to seamlessly incorporate Generative AI into your existing systems, ensuring optimal results.
1. Seamless Integration: Incorporating Generative AI into Existing Frameworks
Begin by conducting a thorough analysis of your current sentiment analysis system. Identify key touchpoints where Generative AI customer sentiment analysis can enhance accuracy and understanding.
Once identified, adopt a phased approach to integration. Prioritize areas with high impact and low disruption potential. Collaborate with your IT team to ensure compatibility and address any technical challenges. A gradual integration minimizes the learning curve and allows your team to adapt smoothly to the enhanced capabilities of Generative AI for customer service sentiment analysis.
2. Tailored Solutions: Training Generative AI Models for Specific Industries
Tailoring Generative AI models to the specific language and context of your industry is crucial for accurate customer sentiment analysis using generative AI. Begin by curating a dataset that reflects the diversity of languages used within your sector.
Invest in training your Generative AI chatbot models on this industry-specific dataset. Fine-tune the models to recognize domain-specific jargon, slang, and sentiment expressions. Regularly update the training data to keep the model current with evolving industry trends. This tailored approach ensures that your AI powered customer sentiment analysis is attuned to the intricacies of your business environment, enhancing the benefits of customer sentiment analysis.
3. Collaborative Approach: Engaging Data Scientists and AI Experts for Successful Implementation
The successful implementation of Generative AI requires a collaborative effort between your business teams and skilled data scientists or AI experts. Foster open communication channels between domain experts and technical teams to ensure a shared understanding of the objectives and challenges in customer sentiment analysis using gen ai.
Engage data scientists to oversee the training and deployment of Generative AI models. Leverage their expertise to fine-tune algorithms, optimize performance, and troubleshoot any issues that may arise. A collaborative approach ensures that the implementation aligns with your business goals and maximizes the potential of Generative AI customer sentiment analysis.
Conclusion
Integrating Generative AI into customer sentiment analysis marks a significant stride toward building more intelligent systems. As we navigate the complex landscape of customer feedback, harnessing the power of Generative AI text analysis not only enhances the accuracy and efficiency of sentiment analysis chatgpt but also opens new avenues for understanding subtle expressions and evolving language patterns.
The ability to generate contextually relevant responses positions businesses at the forefront of customer experience innovation. This, coupled with the capacity to adapt to the ever-changing nature of customer sentiment, further enhances their position in the evolving landscape. By embracing this fusion of technology, organizations can not only decipher sentiments more comprehensively but also proactively address customer needs, fostering stronger connections and enhancing overall satisfaction with customer sentiment analysis tools.
For more insights on how AI can revolutionize customer service, check out this article on How ChatGPT Can Help in Customer Service.
About Wizr AI
Wizr AI is an Advanced Enterprise AI Platform that empowers businesses to build Autonomous AI Agents, AI Assistants, and AI Workflows, enhancing enterprise productivity and customer experiences. Our CX Control Room leverages Generative AI to analyze insights, predict escalations, and optimize workflows. CX Agent Assist AI delivers Real-Time Agent Assist, boosting efficiency and resolution speed, while CX AutoSolve AI automates issue resolution with AI-Driven Customer Service Automation. Wizr Enterprise AI Platform enables seamless Enterprise AI Workflow Automation, integrating with data to build, train, and deploy AI agents, assistants, and applications securely and efficiently. It offers pre-built AI Agents for Enterprise across Sales & Marketing, Customer Support, HR, ITSM, domain-specific operations, Document Processing, and Finance.
Experience the future of enterprise productivity—request a demo of Wizr AI today.
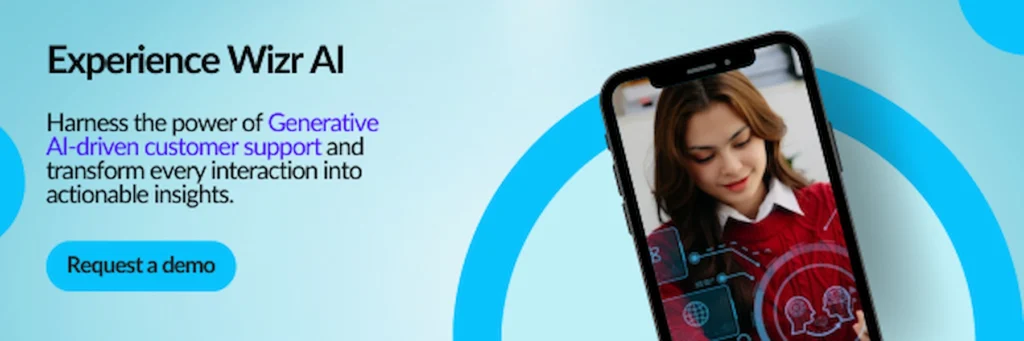